Why Most Investment in AI Will Underdeliver or Fail
People and businesses are obsessed with the potential of AI, but 80% of AI projects will fail—and it's not down to want or enthusiasm. While AI is permeating every industry and sector, the issue lies in businesses not adequately readying themselves for this technological change. Boston Consulting Group reports that one in three companies globally […] The post Why Most Investment in AI Will Underdeliver or Fail appeared first on Unite.AI.
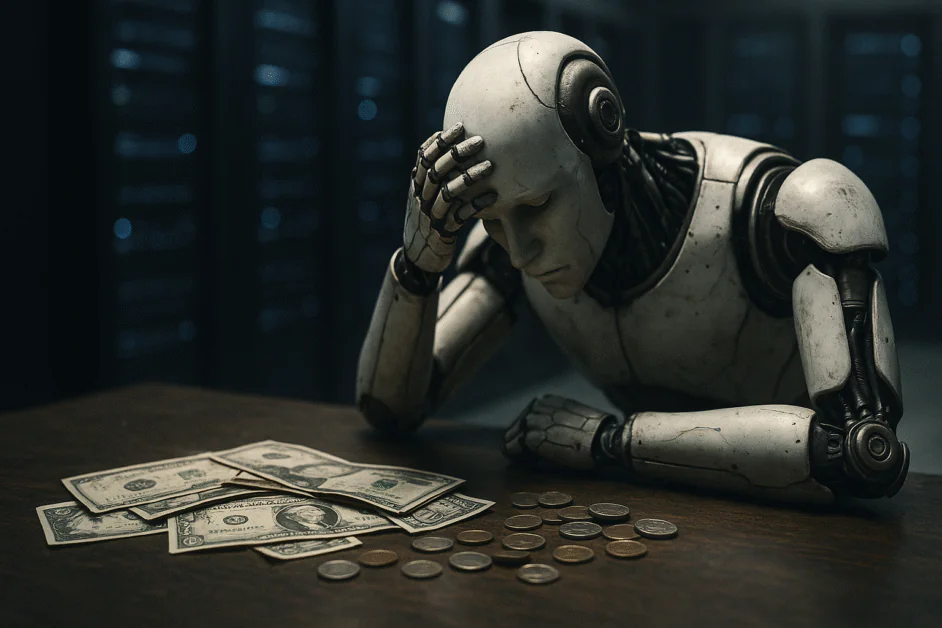

People and businesses are obsessed with the potential of AI, but 80% of AI projects will fail—and it's not down to want or enthusiasm.
While AI is permeating every industry and sector, the issue lies in businesses not adequately readying themselves for this technological change.
Boston Consulting Group reports that one in three companies globally plans to spend over $25 million on AI. Therefore, millions of dollars will be wasted if businesses continue to dive into AI solutions without planning ahead.
However, with strong change management initiatives and a system to support new innovation and measurable KPIs, businesses can turn the tide on their AI's success story.
Let's dive into the three top reasons why AI initiatives fail .
Putting Technology First and Business Second
Hundreds of reports and studies, especially regarding generative AI, show the speed and impressive intellectual dexterity of AI algorithms and programs.
A lot of innovation has gone into AI, leading companies to want to jump in feet-first and invest in leveraging cutting-edge prototypes. However, the risk is that they can spend millions of dollars on a solution that results in an unclear business goal or no measurable impact.
In fact, Gartner predicts that at least 30% of generative AI projects will be abandoned by the end of 2025 due to poor data quality, inadequate risk controls, and escalating costs or unclear business value.
Poor data is a particular hurdle that most businesses fail to overcome, especially when it comes to maximizing the efficiency and effectiveness of AI solutions. Siloed data is among the most prominent issues, and is a business problem that can’t be ignored. Teams can end up wasting hours trying to chase down missing information crucial to strategic decision-making.
And it’s not just teams that are undermined, but tools as well. Machine learning models, for example, are not able to perform properly when data is disconnected and riddled with errors.
To ensure a positive ROI on the investment, and before any technical work begins, organizations must identify the specific business problems the AI solution is intended to solve. This includes setting measurable KPIs and goals, such as cost reduction, revenue increase, or efficiency improvements like cutting down the time it takes to retrieve data.
Specifically, the business strategy should come first, and the technology implementation follows accordingly. Ultimately, technological solutions should serve as a means of driving business outcomes. Moreover, the business need is essentially the backbone of AI and other technology implementations.
For example, a logistics company that wants to leverage AI might lay out measurable goals for their AI software to optimize demand forecasting and enhance fleet management, reducing the number of underused trucks by 25% in the first six months and helping them to increase profits by 5%.
Businesses need measurable goals to consistently check that the AI is not only improving efficiency but that it's quantifiable. This is essential when explaining to company stakeholders that the expensive AI gamble was not only worth it, but they have the data to prove it.
Overambitious AI Implementation
AI’s promise to revolutionize everything is consistently reiterated in the media and is often misrepresented as a silver bullet. This can instill a sense of false confidence in business leaders, leading them to believe they can leverage new AI systems and integrate them all into business processes simultaneously.
However, overambitious attempts to solve a problem in one fell swoop usually lead to failure. Instead, businesses should start small and scale strategically for better results.
For instance, success has been shown on a large scale with Walmart, which introduced machine learning algorithms incrementally to optimize inventory management. The result? A 30% reduction in overstock inventory and a 20% increase in on-shelf availability.
To help with this, businesses should adapt to a ‘zone to win' framework for AI implementation, a proven methodology that helps teams understand that they must balance current operation with future innovation.
The framework divides business activities into four zones: performance, productivity, incubation, and transformation. AI can't disrupt everything at once, and the incubation zone creates a dedicated space for experimenting with AI technologies without disrupting core business.
For example, this is how the ‘zone to win' framework could apply to a cold storage logistics company implementing AI:
- Performance zone: The company’s core business operations, such as warehouse scheduling and goods deployment are key to generating revenue. KPIs around improving warehouse efficiency to slash dwell times and increase deliveries are priorities.
- Productivity zone: Here, internal processes are addressed to boost efficiency and cut costs like detention charges by integrating data science capabilities such as predictive analytics and real-time analytics tools.
- Incubation zone: The company dedicates time to pilot data-driven tools in certain warehouses, allowing teams to determine which innovations could become future revenue streams.
- Transformation zone: This is where the company expands its digital transformation to an organization-wide scale, following a comprehensive digital infrastructure that ensures recurring business outcomes.
The framework helps leadership make decisions about resource allocation between maintaining current operations and investing in AI-driven future capabilities. This awareness helps to avoid the issue and inevitable failure when AI investments are spread too thinly across too many departments and processes.
Lack of User Adoption
Companies are rushing to leverage all the benefits AI and machine learning offer without first considering the people using them. Even the most sophisticated AI solutions fail if end users don't understand the technology—it all hangs on trust and comprehensive training.
The vital underlying factor to integrating AI is operationalizing it. That means ensuring AI tools are plugged into workflows and are made mainstream to business processes.
Other work tools, such as CRMs, optimize and control an entire process from start to finish. This makes training easy as each step of the process can be shown and explained. However, generative AI operates at a more granular ‘task level' rather than encompassing entire processes. It can be used sporadically within various steps of different methods; rather than supporting a complete workflow, each user might apply the AI slightly differently for their specific tasks.
Ruth Svensson, a partner at KPMG UK, told Forbes: “Because generative AI operates at a task level rather than at a process level, you can't see the training gaps as easily.” As a result, employees may be using the AI tool without understanding how it fits into the broader business goals, leading to hidden training gaps. These gaps might include a lack of understanding of how to leverage the AI's capabilities fully, how to interact with the system effectively, or how to ensure the data it generates is used correctly.
In this case, effective change management becomes crucial for user adoption. Change management allows organizations to ensure their employees are not just adopting the new technology but also grasping its full implications for their tasks and business processes.
Without proper change management, companies will miss the mark when it comes to user adoption of AI tools while running the risk of exacerbating technology gaps which are a slipper slope to more inefficiencies, mistakes, and a failure to maximize the potential of the AI solution.
For change management initiatives to work, they need a designated qualified leadership team to spearhead the movement. Leaders must identify training gaps at the task level and provide or organize tailored training for employees based on the specific tasks they will be using AI for.
The idea is to empower and encourage employees to have greater comprehension and confidence in the new system. Only then will understanding and acceptance come, leading to businesses enjoying widespread adoption and better application of the technology.
It's clear that AI is the defining technology of this decade, but without operationalization, its impact will continue to be wasted. By upgrading change management initiatives, implementing AI initiatives slowly, and using measurable KPIs, businesses won't just be spending on AI; they'll be profiting from it.
The post Why Most Investment in AI Will Underdeliver or Fail appeared first on Unite.AI.