From Code to Robots: The Top AI Trends Transforming Business and Life
Artificial intelligence is no longer a concept of the distant future – it’s here, evolving at a rapid pace and reshaping industries in real time. From healthcare to entertainment, AI’s influence is everywhere, sparking innovation, efficiency, and even ethical debates. But with so much happening at once, where exactly is the industry heading? To make […] The post From Code to Robots: The Top AI Trends Transforming Business and Life appeared first on TOPBOTS.
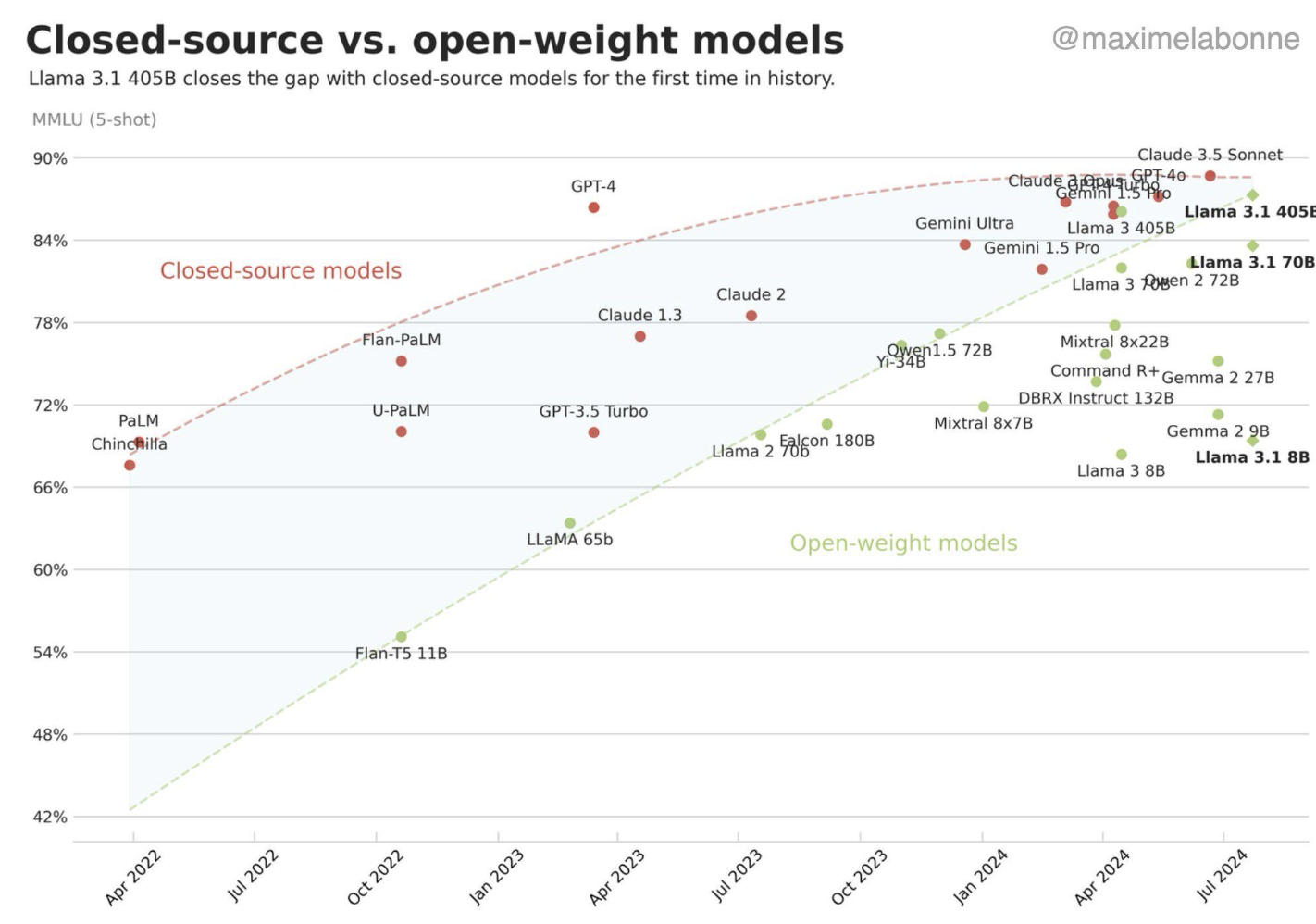
Artificial intelligence is no longer a concept of the distant future – it’s here, evolving at a rapid pace and reshaping industries in real time. From healthcare to entertainment, AI’s influence is everywhere, sparking innovation, efficiency, and even ethical debates. But with so much happening at once, where exactly is the industry heading? To make sense of the chaos, we’ve curated a list of the most compelling trends that are not only making headlines but are also set to define the next chapter of AI’s journey. These trends highlight the groundbreaking advancements pushing the boundaries of what AI can achieve.
In this article, we’ll explore the top 10 key trends shaping the future of AI, from the rise of multimodal systems that process text, images, video, and audio, to the increasing demand for smaller, more efficient models. We’ll also delve into the growing importance of open-source AI, the emergence of autonomous agents, and the expanding role of AI in sectors like coding, gaming, and humanoid robotics. Buckle up for a deep dive into how AI is transforming our world – one breakthrough at a time.
If this in-depth educational content is useful for you, subscribe to our AI mailing list to be alerted when we release new material.
The Top 10 AI Trends to Watch
As AI continues to evolve, several key trends are emerging that highlight the most exciting and transformative directions in the industry. From innovations in model architecture to AI applications in everyday technology, these trends offer a glimpse into the future of what AI will be capable of. Let’s dive into the ten trends currently driving the AI landscape forward.
1. Multimodal AI
Large Language Models (LLMs) earned their name because they were originally designed to process text data – language, in its various forms. But as the world around us is inherently multimodal, the next logical step has been to create AI models that can process multiple types of data simultaneously. This shift towards multimodality has led to the development of models like OpenAI’s GPT-4, Anthropic’s Claude-3.5, and Google’s Gemini models, which were designed as multimodal from the outset. These models are not only capable of understanding and generating text but can also interpret images, analyze audio, and even process videos, opening the door to a new universe of possibilities.
Multimodal AI enables a broad set of applications across industries. For instance, these models can provide more dynamic customer support by interpreting images sent by users, generate creative content like video scripts or music based on a combination of visual and textual inputs, or enhance accessibility tools by converting text into sound and vice versa. Additionally, multimodal capabilities strengthen AI models by exposing them to diverse data types, enriching their learning process and improving overall accuracy and adaptability. This evolution toward multimodality is driving more powerful and versatile AI systems, setting the stage for groundbreaking applications in areas like education, healthcare, and entertainment.
2. Small models
As the race for AI dominance continues, a significant trend is the development of smaller, more efficient models that can deliver high-quality results without the need for massive computational resources. Recent examples include OpenAI’s GPT-4o Mini, Microsoft Azure’s Phi-3 models, Apple’s On-Device models, Meta’s LLaMA 3 8B, and Google’s Gemma-7B. These smaller models are designed to offer robust performance while using far fewer resources, making them suitable for a range of applications, including those that could run directly on mobile devices or edge hardware.
The drive to create smaller models is fueled by several factors. First, they consume less power and require lower computational costs, which is especially important for enterprises looking to implement AI solutions at scale in an energy-efficient manner. Second, some of these models, like Apple’s On-Device models, are optimized to run directly on smartphones and other portable devices, enabling AI capabilities such as real-time translation, voice recognition, and enhanced user experiences without relying on cloud processing. By focusing on efficiency and accessibility, these small models are helping to democratize AI, making powerful technologies available to more users and industries, while reducing the infrastructure burden typically associated with larger models.
3. Open source models
Open-source LLMs have become a cornerstone of democratizing AI, providing unrestricted accessibility and empowering developers across different sectors and skill levels. However, there is ongoing debate about what truly constitutes an “open-source” model. Recently, The Open Source Initiative (OSI) – a key body defining open-source standards – released a new definition, stating that for an AI system to be considered open source, it must allow anyone to use it for any purpose without needing permission. Moreover, researchers should have full access to inspect its components and understand how the system works, including details about the training data. By this standard, many AI models that are commonly referred to as “open-source” may not fully qualify, as they often lack transparency around their training data and impose some restrictions on commercial use. As a result, these models are better described as “open-weight” models, which offer open access to their model weights but with certain limitations.
The open-weight models have made impressive strides, narrowing the gap with the performance of leading closed models. Meta’s release of LLaMA 3.1 405B set a new benchmark, outperforming proprietary models like GPT-4o and Claude 3.5 Sonnet in some key areas. Other notable open-weight models include the Mistral models, Grok models from Elon Musk’s xAI, and Google’s Gemma models. Open-source aproaches are crucial for fostering transparency and ethical AI development, as greater scrutiny of the code can help uncover biases, bugs, and security vulnerabilities. However, there are valid concerns about the potential misuse of open-source AI to generate disinformation and other harmful content. The challenge moving forward is finding a balance between democratizing AI development and ensuring responsible, ethical use of these powerful technologies.
4. Agentic AI
Agentic AI represents a major shift in the capabilities of artificial intelligence, moving from reactive systems to proactive, autonomous agents. Unlike traditional AI models, which operate by responding to specific user inputs or following predetermined rules, AI agents are designed to independently assess their environment, set goals, and execute actions without continuous human direction. This autonomy allows them to decide what steps to take to complete complex tasks that cannot be done in a single step or with just one tool. In essence, Agentic AI is capable of making decisions and taking action in pursuit of specific objectives, revolutionizing what AI can achieve.
These advanced agents open the door to applications at incredibly high-performance levels. One compelling example is AI Scientist, an agentic system that guides large language models to generate novel ideas for AI research, write code to test those ideas, and even produce research papers based on the findings. Another fascinating application is TransAgents, which uses a multi-agent workflow to translate Chinese novels into English. Here, different LLMs (or instances of the same model) act as agents in roles like translator or localization specialist, checking and revising each other’s work. As a result, TransAgents produce translations at about the same quality level as professional translators.
As agentic AI evolves, we are likely to see even more applications across diverse sectors, pushing the boundaries of what AI can achieve independently.
5. Customized Enterprise AI Models
While massive, general-purpose models like GPT-4 and Gemini have captured much of the public’s attention, their utility for business-specific applications may be limited. Instead, the future of AI in the enterprise space is increasingly leaning toward smaller, purpose-driven models designed to address niche use cases. Businesses are demanding AI systems that cater to their specific needs, and these tailored models are proving to offer greater staying power and long-term value.
Building an entirely new AI model from scratch, though possible, is often prohibitively expensive and resource-intensive for most organizations. Instead, many opt to customize existing models, either by tweaking their architecture or fine-tuning them with domain-specific datasets. This approach is more cost-effective than building from the ground up and allows companies to avoid the recurring costs of relying on API calls to a public LLM.
Recognizing this demand, providers of general-purpose models are adapting. For example, OpenAI now offers fine-tuning options for GPT-4o, enabling businesses to optimize the model for higher accuracy and performance in specific applications. Fine-tuning allows for adjusting the model’s tone, structure, and responsiveness, making it better suited for complex, domain-specific instructions.
There are already success stories emerging from this trend. Cosine’s Genie, an AI software engineering assistant built on a fine-tuned version of GPT-4o, has delivered state-of-the-art results in bug resolution, feature development, and code refactoring. Similarly, Distyl, another customized version of GPT-4o, has excelled in tasks like query reformulation, intent classification, and SQL generation, proving the power of tailored AI for technical tasks. This is just the beginning – OpenAI and other companies are committed to expanding customization options to meet growing enterprise demand.
Custom generative AI tools can be developed for nearly any business scenario, whether it’s customer support, supply chain management, or legal document review. Industries like healthcare, finance, and law, with their unique terminology and workflows, stand to benefit immensely from these tailored AI systems, which are quickly becoming indispensable for companies seeking precision and efficiency.
6. Retrieval-Augmented Generation
One of the major challenges facing generative AI models is the issue of “hallucinations” – instances where the AI generates responses that sound convincing but are factually incorrect. This has been a significant barrier for businesses looking to integrate AI into mission-critical or customer-facing operations, where such errors can lead to serious consequences. Retrieval-augmented generation (RAG) has emerged as a promising solution to this problem, offering a way to enhance the accuracy and reliability of AI outputs. By enabling AI models to pull in real-time information from external databases or knowledge sources, RAG allows models to provide fact-based, up-to-date responses, rather than relying solely on pre-existing internal data.
RAG has profound implications for enterprise AI, particularly in industries that demand precision and up-to-the-minute accuracy. For example, in healthcare, AI systems using RAG can retrieve the latest research or clinical guidelines to support medical professionals in decision-making. In customer service, RAG-enabled AI chatbots can access a company’s knowledge base to resolve customer issues with accuracy and relevance. Similarly, legal firms can use RAG to enhance document review by pulling in relevant case law or statutes on the fly, reducing the risk of errors. RAG not only helps curb the hallucination problem but also allows models to remain lightweight, as they don’t need to store all potential knowledge internally. This leads to faster performance and reduced operational costs, making AI more scalable and trustworthy for enterprise applications.
7. Voice Assistants
Generative AI is revolutionizing the way we interact with voice assistants, making conversations more fluid, natural, and responsive. OpenAI’s GPT-4o with voice capabilities, recently demoed, promises a significant leap in conversational AI. With an average response time that closely mirrors human dialogue, it supports more dynamic interactions, allowing users to engage in real-time conversations without awkward pauses. Meanwhile, Google is pushing the envelope with its Project Astra, which integrates advanced voice features to create seamless, intuitive conversations between users and AI. These developments signal a major shift in how voice assistants will function in the near future, moving from basic, command-driven interactions to rich, conversational exchanges.
Apple is also stepping up its game, with Siri set to offer more natural responses based on the latest presentation from the company. The improvements are expected to make Siri much more responsive and intuitive, closing the gap between human conversation and AI interaction. This evolution means that soon, we’ll be interacting with AI voice assistants in a way that feels like speaking to a well-informed colleague. Voice assistants could transform how we handle a range of tasks – from scheduling meetings and answering emails to managing smart home systems and even assisting in healthcare by offering real-time symptom analysis. While we may not rely solely on voice, the ability to seamlessly switch to voice interaction will soon become the standard, making AI assistants more adaptable and user-friendly across a variety of contexts.
8. AI for Coding
The intersection of AI and software development is experiencing rapid growth, with a surge of funding highlighting the sector’s potential. Recent investments in companies like Magic, an AI startup focusing on code generation, which raised a staggering $320 million, and Codeium, an AI-powered code acceleration platform that secured $150 million in Series C funding, underscore the excitement in this space. Additionally, Cosine, previously noted for its fine-tuned GPT-4o model, secured $2.5 million in funding for its AI developer, which has demonstrated the ability to outperform human coders in tasks such as debugging and feature development. These investments indicate a booming interest in AI-driven coding solutions, as businesses seek ways to improve the efficiency and effectiveness of their software development pipelines.
Generative AI is already transforming the coding process by automating tasks like code generation, debugging, and refactoring, significantly reducing the time and effort required for developers to complete projects. For instance, platforms like GitHub Copilot have been shown to boost developer productivity by up to 55% by suggesting code snippets, identifying errors, and offering real-time coding assistance. Use cases for AI in coding extend beyond just writing code – AI can help streamline testing, automate documentation, and even optimize performance. This increased speed and efficiency not only benefits individual developers but also entire development teams, allowing them to focus on more complex tasks while AI handles repetitive and time-consuming aspects of the coding process. With continued advancements, AI-powered coding tools are set to become an integral part of modern software development.
9. Humanoid Robots
Humanoid robots are rapidly gaining momentum as advancements in robotics and AI drive their development for various applications. Designed to mimic human physical capabilities, these robots are developing new functionalities to be used in industries such as manufacturing, warehousing, and logistics, where their flexibility allows them to handle tasks that require precision, dexterity, and adaptability. Companies like Tesla, with its Optimus robot, Figure Robotics, Agility Robotics, and 1X are leading the charge in this growing sector.
However, the applications for humanoid robots are not limited to factories and warehouses. 1X’s Neo and Weave’s Issac robots are designed to become home assistants, with Weave’s recently introduced robot butler being able to help with everyday chores such as cleaning and organizing the home. These robots are also showing promise in caregiving, where they could assist elderly individuals with daily activities or provide basic companionship.
As advancements continue, humanoid robots are likely to become more common in both professional and personal spaces, supporting humans with tasks that require physical interaction in our everyday environments.
10. AI in Gaming
AI is transforming the gaming industry in profound ways, with generative AI leading the charge by enabling the automatic creation of complex assets like 3D objects, characters, and even entire environments. Instead of painstakingly designing each object or landscape by hand, developers can now use AI models to generate lifelike or fantastical elements at scale, speeding up the production process and enhancing creativity. For example, AI-powered tools can design diverse terrain, buildings, and non-playable characters (NPCs) that react dynamically to players’ actions, making worlds more immersive and reducing the workload for game designers.
A particularly exciting development comes from Google’s new AI gaming engine, which has demonstrated the ability to recreate classic games like DOOM, as well as potentially any other game. This technology could revolutionize how games are developed and remastered, offering new ways for developers and fans alike to experience their favorite titles. By using AI to recreate the mechanics, graphics, and even storylines of iconic games, this technology not only preserves gaming history but also opens the door for new iterations and modifications. The implications are enormous: generative AI could give rise to personalized games, where players can influence everything from story arcs to the design of their game world, resulting in highly tailored and unique experiences.
As these technologies advance, we may see a future where AI helps both indie developers and large studios produce highly detailed, immersive games faster and at lower cost, while allowing for unprecedented creativity and customization.
Shaping the Future of AI: What’s Next?
The rapid advancements in AI across various domains are redefining what’s possible in both enterprise and personal applications. Each of the discussed trends – whether it’s the rise of agentic AI, the fine-tuning of enterprise models, or the growing role of AI in software development – points toward a future where AI becomes increasingly embedded in our daily lives. As AI continues to evolve, it will not only enhance productivity and creativity but also open up new ethical considerations and challenges, especially as more industries embrace these technologies.
The future of AI is both exciting and complex. Whether it’s reshaping industries like manufacturing, healthcare, and gaming, or revolutionizing personal assistants and enterprise workflows, AI is poised to play a central role in the way we live and work. As these trends mature, the key challenge will be ensuring that AI’s development remains balanced, ethical, and beneficial to society at large.
Enjoy this article? Sign up for more AI updates.
We’ll let you know when we release more summary articles like this one.
The post From Code to Robots: The Top AI Trends Transforming Business and Life appeared first on TOPBOTS.