Ensuring Resilient Security for Autonomous AI in Healthcare
The raging war against data breaches poses an increasing challenge to healthcare organizations globally. As per current statistics, the average cost of a data breach now stands at $4.45 million worldwide, a figure that more than doubles to $9.48 million for healthcare providers serving patients within the United States. Adding to this already daunting issue […] The post Ensuring Resilient Security for Autonomous AI in Healthcare appeared first on Unite.AI.
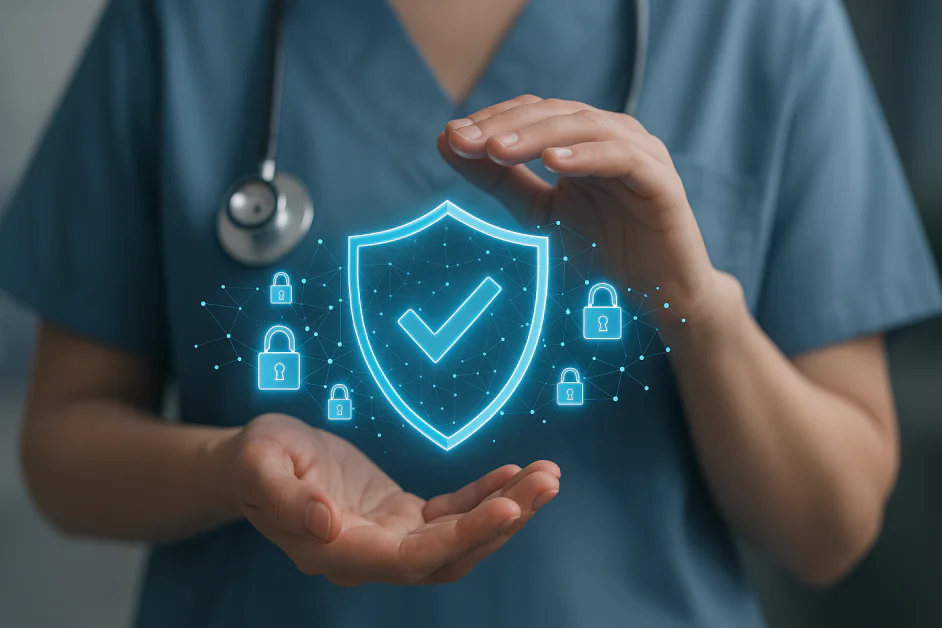

The raging war against data breaches poses an increasing challenge to healthcare organizations globally. As per current statistics, the average cost of a data breach now stands at $4.45 million worldwide, a figure that more than doubles to $9.48 million for healthcare providers serving patients within the United States. Adding to this already daunting issue is the modern phenomenon of inter- and intra-organizational data proliferation. A concerning 40% of disclosed breaches involve information spread across multiple environments, greatly expanding the attack surface and offering many avenues of entry for attackers.
The growing autonomy of generative AI brings an era of radical change. Therefore, with it comes the pressing tide of additional security risks as these advanced intelligent agents move out of theory to deployments in several domains, such as the health sector. Understanding and mitigating these new threats is crucial in order to up-scale AI responsibly and enhance an organization’s resilience against cyber-attacks of any nature, be it owing to malicious software threats, breach of data, or even well-orchestrated supply chain attacks.
Resilience at the design and implementation stage
Organizations must adopt a comprehensive and evolutionary proactive defense strategy to address the increasing security risks caused by AI, especially inhealthcare, where the stakes involve both patient well-being as well as compliance with regulatory measures.
This requires a systematic and elaborate approach, starting with AI system development and design, and continuing to large-scale deployment of these systems.
- The first and most critical step that organizations need to undertake is to chart out and threat model their entire AI pipeline, from data ingestion to model training, validation, deployment, and inference. This step facilitates precise identification of all potential points of exposure and vulnerability with risk granularity based on impact and likelihood.
- Secondly, it is important to create secure architectures for the deployment of systems and applications that utilize large language models (LLMs), including those with Agentic AI capabilities. This involves meticulously considering various measures, such as container security, secure API design, and the safe handling of sensitive training datasets.
- Thirdly, organizations need to understand and implement the recommendations of various standards/ frameworks. For example, adhere to the guidelines laid down by NIST's AI Risk Management Framework for comprehensive risk identification and mitigation. They could also consider OWASP's advice on the unique vulnerabilities introduced by LLM applications, such as prompt injection and insecure output handling.
- Moreover, classical threat modeling techniques also need to evolve to effectively manage the unique and intricate attacks generated by Gen AI, including insidious data poisoning attacks that threaten model integrity and the potential for generating sensitive, biased, or inappropriately produced content in AI outputs.
- Lastly, even after post-deployment, organizations will need to stay vigilant by practicing regular and stringent red-teaming maneuvers and specialized AI security audits that specifically target sources such as bias, robustness, and clarity to continually discover and mitigate vulnerabilities in AI systems.
Notably, the basis of creating strong AI systems in healthcare is to fundamentally protect the entire AI lifecycle, from creation to deployment, with a clear understanding of new threats and an adherence to established security principles.
Measures during the operational lifecycle
In addition to the initial secure design and deployment, a robust AI security stance requires vigilant attention to detail and active defense across the AI lifecycle. This necessitates for the continuous monitoring of content, by leveraging AI-driven surveillance to detect sensitive or malicious outputs immediately, all while adhering to information release policies and user permissions. During model development and in the production environment, organizations will need to actively scan for malware, vulnerabilities, and adversarial activity at the same time. These are all, of course, complementary to traditional cybersecurity measures.
To encourage user trust and improve the interpretability of AI decision-making, it is essential to carefully use Explainable AI (XAI) tools to understand the underlying rationale for AI output and predictions.
Improved control and security are also facilitated through automated data discovery and smart data classification with dynamically changing classifiers, which provide a critical and up-to-date view of the ever-changing data environment. These initiatives stem from the imperative for enforcing strong security controls like fine-grained role-based access control (RBAC) methods, end-to-end encryption frameworks to safeguard information in transit and at rest, and effective data masking techniques to hide sensitive data.
Thorough security awareness training by all business users dealing with AI systems is also essential, as it establishes a critical human firewall to detect and neutralize possible social engineering attacks and other AI-related threats.
Securing the future of Agentic AI
The basis of sustained resilience in the face of evolving AI security threats lies in the proposed multi-dimensional and continuous method of closely monitoring, actively scanning, clearly explaining, intelligently classifying, and stringently securing AI systems. This, of course, is in addition to establishing a widespread human-oriented security culture along with mature traditional cybersecurity controls. As autonomous AI agents are incorporated into organizational processes, the necessity for robust security controls increases. Today’s reality is that data breaches in public clouds do happen and cost an average of $5.17 million , clearly emphasizing the threat to an organization’s finances as well as reputation.
In addition to revolutionary innovations, AI's future depends on developing resilience with a foundation of embedded security, open operating frameworks, and tight governance procedures. Establishing trust in such intelligent agents will ultimately decide how extensively and enduringly they will be embraced, shaping the very course of AI's transformative potential.
The post Ensuring Resilient Security for Autonomous AI in Healthcare appeared first on Unite.AI.