AWS Glue DataBrew
Service Overview Service Name: AWS Glue DataBrew Logo: Tagline or One-Line Description: "AWS Glue DataBrew: Clean, Prepare, and Transform Your Data Visually, Without Writing Code." Key Features Top Features: Automated Data Profiling. Secure and Reliable. Scalable Data Handling. Technical Specifications: Supported Regions : Available in most AWS regions worldwide. Dataset Profiling Limits: Up to 20 million rows or 10 GB per profiling session. Security : Full integration with AWS IAM for role-based access and resource permissions. Data Sources : Compatible with Amazon S3, Redshift, RDS, and other JDBC-supported data sources. Use Cases Real-Life Applications: AWS Glue DataBrew Cleaning Customer Data : Fix issues like missing names or incorrect dates in customer data for better analysis. Preparing Data for Reports : Format and clean data so it's ready to be used in business reports. Combining Data from Different Sources: Merge data from Amazon S3 and RDS to create a single dataset for analysis. Pricing Model Pricing Overview: AWS Glue DataBrew uses a pay-as-you-go pricing model.Pricing based on Data Processing Charges. Compute Resource Usage. Data Profiling Sessions. Automated Job Scheduling. Comparison with Similar Services Competitors or Alternatives: Google Cloud Dataprep (Non-AWS):Provides a no-code interface for data cleaning but focuses on Google Cloud integration. Microsoft Power Query (Non-AWS):Ideal for small-scale, desktop-based data preparation, integrating seamlessly with Excel and Power BI. Azure Data Factory (AWS Alternative):Offers data transformation within the Azure ecosystem but has a steeper learning curve, suited for advanced users. Apache NiFi (Non-AWS):Open-source tool for data routing and transformation but needs complex configurations. Benefits and Challenges Advantages: No-Code Interface, Scalability, Over 250 Pre-Built Transformations, Automated Data Profiling, Security and Compliance. Limitations or Challenges: Cost for Large Datasets, Complexity for Advanced Data Processing, Dependency on AWS Services, Limited Output Formats. Real-World Example or Case Study Case Study: Coca-Cola Company. Example: Coca-Cola uses AWS Glue DataBrew to simplify and automate their data preparation processes, which are essential for generating business insights across various markets.

Service Overview
Service Name: AWS Glue DataBrew
Logo:
Tagline or One-Line Description: "AWS Glue DataBrew: Clean, Prepare, and Transform Your Data Visually, Without Writing Code."Key Features
Top Features:
Automated Data Profiling.
Secure and Reliable.
Scalable Data Handling.
Technical Specifications:
Supported Regions : Available in most AWS regions worldwide.
Dataset Profiling Limits: Up to 20 million rows or 10 GB per profiling session.
Security : Full integration with AWS IAM for role-based access and resource permissions.
Data Sources : Compatible with Amazon S3, Redshift, RDS, and other JDBC-supported data sources.Use Cases
Real-Life Applications: AWS Glue DataBrew
Cleaning Customer Data : Fix issues like missing names or incorrect dates in customer data for better analysis.
Preparing Data for Reports : Format and clean data so it's ready to be used in business reports.
Combining Data from Different Sources: Merge data from Amazon S3 and RDS to create a single dataset for analysis.Pricing Model
Pricing Overview: AWS Glue DataBrew uses a pay-as-you-go pricing model.Pricing based on
Data Processing Charges.
Compute Resource Usage.
Data Profiling Sessions.
Automated Job Scheduling.Comparison with Similar Services
Competitors or Alternatives:
Google Cloud Dataprep (Non-AWS):Provides a no-code interface for data cleaning but focuses on Google Cloud integration.
Microsoft Power Query (Non-AWS):Ideal for small-scale, desktop-based data preparation, integrating seamlessly with Excel and Power BI.
Azure Data Factory (AWS Alternative):Offers data transformation within the Azure ecosystem but has a steeper learning curve, suited for advanced users.
Apache NiFi (Non-AWS):Open-source tool for data routing and transformation but needs complex configurations.Benefits and Challenges
Advantages: No-Code Interface, Scalability, Over 250 Pre-Built Transformations, Automated Data Profiling, Security and Compliance.
Limitations or Challenges: Cost for Large Datasets, Complexity for Advanced Data Processing, Dependency on AWS Services, Limited Output Formats.Real-World Example or Case Study
Case Study: Coca-Cola Company.
Example: Coca-Cola uses AWS Glue DataBrew to simplify and automate their data preparation processes, which are essential for generating business insights across various markets.
What's Your Reaction?
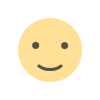
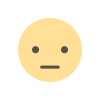
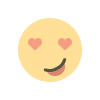
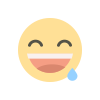
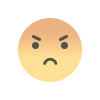
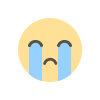
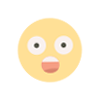