Implementing Advanced Analytics in Real Estate: Using Machine Learning to Predict Market Shifts
When it comes to the real estate industry, we have traditionally relied on local economic indicators, insights from personal networks, and comparisons of historical data to deliver market evaluations. Machine learning has disrupted many industries over the past few years, but the effects it has had in the real estate market fluctuation forecasting area have […] The post Implementing Advanced Analytics in Real Estate: Using Machine Learning to Predict Market Shifts appeared first on Unite.AI.
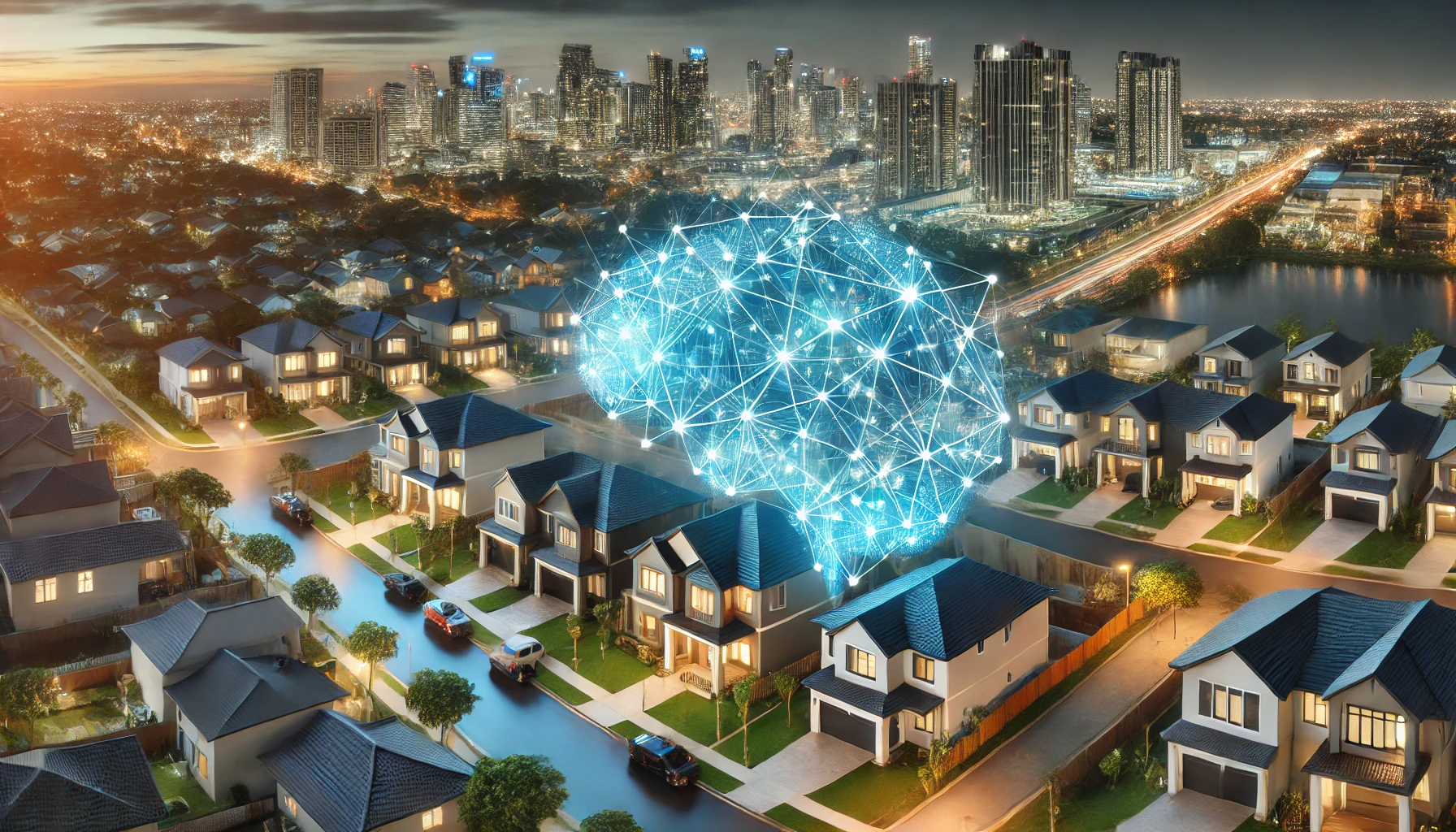
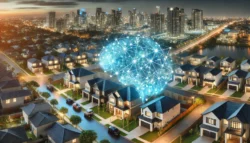
When it comes to the real estate industry, we have traditionally relied on local economic indicators, insights from personal networks, and comparisons of historical data to deliver market evaluations. Machine learning has disrupted many industries over the past few years, but the effects it has had in the real estate market fluctuation forecasting area have been nothing short of transformative. Drawing from my experience at Kalinka Group and Barnes International Moscow, I’ve witnessed how deep analytics has allowed us to explore massive datasets, uncover hidden patterns, and unlock predictive insights previously unimaginable. From 2025 onwards, machine learning will no longer be a utility but a strategic advantage in how real estate is approached.
The Basics of Predictive Analytics in Real Estate
Traditional real estate market analytics methods are being replaced by advanced algorithms capable of analyzing thousands of variables at once, such as property size, location, and comparable sales, which were the focus in the pre-machine learning era. Today’s variables, now addressed by machine learning, include everything from social media sentiment to infrastructure development plans, demographic shifts, neighborhood walkability scores, climate change impacts, and proximity to cultural hubs or transit lines.
For example, the team at Barnes International Moscow successfully predicted neighborhood appreciation rates using machine learning models, an accomplishment that was once unimaginable. These models synthesize data from a variety of sources, ranging from key local economic indicators to online consumer behavior, providing insights far beyond the scope of traditional human analysis.
Data Sources and Integration Challenges
Machine learning thrives on diverse qualitative data, requiring a strong data infrastructure to gather and integrate information from various sources. At Kalinka Group, we designed a roadmap for data acquisition, sourcing information from government property records, pricing trends, real-time listing platforms, and even social media sentiment to understand consumer preferences. We also utilized IoT sensors and smart home devices to measure real-time property performance metrics, enriching our forecasting models to capture everything from supply-demand dynamics to macroeconomic trends and demographic tracking.
Effective data integration is equally important. To ensure the highest degree of accuracy, we implemented rigorous validation checks, transforming raw data into actionable insights while avoiding the pitfalls of “garbage in, garbage out.”
Advanced Analytical Techniques
What excites me most about machine learning is its ability to uncover relationships that traditional statistical methods cannot identify. For instance, at Private Broker, machine learning models revealed how transit line openings influenced property values in nearby neighborhoods.
Other advanced techniques include:
- Gradient Boosting: Combining weak predictive models into a strong, accurate prediction.
- Random Forest Algorithms: Utilizing decision-tree models for enhanced prediction accuracy.
- Natural Language Processing (NLP): Leveraging unstructured data, such as news articles and social media posts, to identify trends and risks.
These approaches provided highly accurate forecasts of market fluctuations, empowering clients to make informed investment decisions.
Practical Applications in Real Estate
Machine learning has transformed nearly every facet of real estate, offering tangible benefits to investors, developers, and property managers.
1. Predicting Market Trends
Machine learning models analyze historical data alongside external variables to forecast market conditions. For example, in a UAE-based project, we utilized ML to analyze economic data, migration patterns, and consumer preferences, uncovering a rising demand for high-end sustainable rental apartments. This insight allowed clients to capitalize on emerging opportunities.
2. Increasing Property Value
Traditional valuation methods relied on comparable sales and broker intuition. Machine learning incorporates hundreds of variables, enabling more accurate and nuanced valuations. At Kalinka Group, Automated Valuation Models (AVMs) provided transparency and speed, winning over clients with data-driven recommendations.
3. Optimizing Commercial Portfolios
Predictive analytics using machine learning forecasts vacancy rates, maintenance costs, and infrastructure impacts, guiding commercial property owners in decision-making. In a Thailand-focused project, machine learning models helped a multinational client rebalance their real estate portfolio, withdrawing from underperforming markets that previously appeared profitable on paper.
4. Identifying Emerging Markets
Machine learning identifies development hotspots by analyzing infrastructure projects, demographic trends, and local economic activities. These insights were pivotal in projects in Cyprus and Turkey, where we advised clients on confidently entering high-growth markets.
Ethical Considerations and Challenges
While machine learning offers immense potential, it also presents ethical and practical challenges.
Data Privacy
Real estate analytics often involves sensitive financial and personal data. At Kalinka Group, we implemented encryption and compliance measures to protect client data while enabling advanced analytics.
Algorithmic Bias
Machine learning models can exhibit bias, leading to discriminatory outcomes. To address this, Barnes International Moscow trained models on diverse datasets, ensuring fairness and transparency in predictive algorithms.
Conclusion
The future of machine learning in real estate is boundless. As quantum computing and more advanced AI models emerge, predictive accuracy will improve further. Real estate professionals are now equipped to anticipate market shifts with unparalleled precision, crafting bespoke strategies for each client and investment. Success will depend on strong data infrastructure, ethical practices, and the ability to combine technological knowledge with professional intuition, ensuring real estate professionals can navigate these transformative times with confidence.
The post Implementing Advanced Analytics in Real Estate: Using Machine Learning to Predict Market Shifts appeared first on Unite.AI.