How Most Organizations Get Data Strategy Wrong — and How to Fix It
Wave by CLEANPNG.DEMYSTIFY DATA STRATEGYHow Most Organizations Get Data & AI Strategy Wrong — and How to Fix ItRedefining Data Strategy to Drive Competitive Advantage with Data, Analytics and AIUnlocking business value from data, analytics, and Artificial Intelligence is a hot topic. Yet, most organizations struggle to monetize existing data or to create new data that advances their thinking. I assert that in most cases, this struggle stems from a lack of effective business strategy design.This is the third installment in a series demystifying data strategy— something that organizations seem to need to become data-driven and remain competitive in today’s digital world. This document serves as a playbook for data strategy, offering a deep and structured exploration by addressing misconceptions, clarifying definitions, presenting actionable frameworks, and illustrating concepts through a detailed example.I am Jens, a business-minded data expert with nearly two decades of experience in implementing data and AI use cases. I help leaders across industries design strategies and foster cultures that unlock the full potential of data and algorithms.tl;drA data strategy is not a standalone supplement to business strategy, nor is it a plan for building data capabilities. It is rather the strategy of a data function, providing services to the internal data customers.A data strategy does not define how an organization creates value with data, analytics and AI. This is the job of the business strategy with data-related strategic choices being an integral part.Misconceptions about data strategy, combined with weak business strategy design, prevent organizations from leveraging data effectively. These issues hinder their ability to gain a competitive advantage.The Playing to Win framework helps organizations with both: embedding data innovation into their business strategy and designing effective data strategies.TABLE OF CONTENTS1 THE FUZZ ABOUT DATA STRATEGY 1.1 Data Can Create Benefits Throughout the Organization 1.2 Organizations Struggle to Become Data-Driven 1.3 The Data Strategy Promise 2 DATA STRATEGY MISCONCEPTIONS 2.1 Misconception #1: A Plan to Build Data and AI Capabilities 2.2 Misconception #2: Approaches and Guidelines How Data is Managed 2.3 Misconception #3: A Supplement to Business Strategy 2.4 Quo Vadis? 3 STRATEGY 3.1 The Playing to Win Strategy Framework 3.2 What Strategy Actually Is 3.3 Plan vs. Strategy: The Typical Misunderstanding 3.4 The Strategic Choice Structuring Process 3.5 What Strategies an Organization Needs 3.6 Reinforcing Rods 3.7 What Strategies an Organization Doesn’t Need 3.8 Strategy Design Teams 3.9 Summarizing Strategy Fundamentals 4 DATA STRATEGY DEFINITION & DESIGN 4.1 Defining Data Strategy 4.2 Data Strategy Choice Cascade 4.3 Data Strategy Design Process 4.4 Step I: Identify Data & AI Demands 4.5 Step II: Collect Challenges 4.6 Step IV: Operational Capabilities & Systems 4.7 Step V: Maturity Assessment 4.8 Step VI: Planning 5 INTEGRATING BUSINESS STRATEGY & DATA 5.1 Data Choices as Part of Your Business Strategy 5.2 Data for Problem Formulation and Possibility Generation 5.3 Data Use Case Validation as Part of Testing 5.4 Concluding Remarks on Business Strategy & Data 6 PRACTICAL EXAMPLE 6.1 Step I: Identify Data & AI Demands 6.2 Step II: Collect Challenges 6.3 Step III: Designing a Data Strategy 6.4 Steps IV-VI: Activating Strategy 7 CONCLUSION REFERENCES1 THE FUZZ ABOUT DATA STRATEGYData, analytics, and Artificial Intelligence (AI) are not new phenomena in business but have grown increasingly relevant over the past decades.A lot of excitement currently exists around generative AI due to its recent impressing advances for generating outputs such as texts or images. When I refer to AI, I do not mean solely generative AI, but the broader context of tools to generate value from data. And these tools include Business Intelligence, Machine Learning or Data Science and are everything but new. They have been around for many years.Where does the persistent interest come from?1.1 Data Can Create Benefits Throughout the OrganizationWhilst data was once mainly relevant to accounting and controlling teams, it is nowadays often success-critical throughout the organization:Marketing uses historical campaign data to identify factors that drive success, enabling more effective allocation of marketing spend.Procurement uses data and analytics to analyze supplier performance, so that disruption can be minimized and cost savings maximized.Sales uses historical data to recommend an optimal point-of-sale portfolio leading to increased revenue.Customer service centers leverage data to predict customer churn, enabling proactive actions that reduce churn rates and drive sustainable revenue growth.Production departments leverage IoT data to prevent machine failures, reducing repair costs and minimizing production downtime.Product development teams enrich existing products and services with digital app


DEMYSTIFY DATA STRATEGY
How Most Organizations Get Data & AI Strategy Wrong — and How to Fix It
Redefining Data Strategy to Drive Competitive Advantage with Data, Analytics and AI
Unlocking business value from data, analytics, and Artificial Intelligence is a hot topic. Yet, most organizations struggle to monetize existing data or to create new data that advances their thinking. I assert that in most cases, this struggle stems from a lack of effective business strategy design.
This is the third installment in a series demystifying data strategy— something that organizations seem to need to become data-driven and remain competitive in today’s digital world. This document serves as a playbook for data strategy, offering a deep and structured exploration by addressing misconceptions, clarifying definitions, presenting actionable frameworks, and illustrating concepts through a detailed example.
I am Jens, a business-minded data expert with nearly two decades of experience in implementing data and AI use cases. I help leaders across industries design strategies and foster cultures that unlock the full potential of data and algorithms.
tl;dr
- A data strategy is not a standalone supplement to business strategy, nor is it a plan for building data capabilities. It is rather the strategy of a data function, providing services to the internal data customers.
- A data strategy does not define how an organization creates value with data, analytics and AI. This is the job of the business strategy with data-related strategic choices being an integral part.
- Misconceptions about data strategy, combined with weak business strategy design, prevent organizations from leveraging data effectively. These issues hinder their ability to gain a competitive advantage.
- The Playing to Win framework helps organizations with both: embedding data innovation into their business strategy and designing effective data strategies.
TABLE OF CONTENTS
1 THE FUZZ ABOUT DATA STRATEGY
1.1 Data Can Create Benefits Throughout the Organization
1.2 Organizations Struggle to Become Data-Driven
1.3 The Data Strategy Promise
2 DATA STRATEGY MISCONCEPTIONS
2.1 Misconception #1: A Plan to Build Data and AI Capabilities
2.2 Misconception #2: Approaches and Guidelines How Data is Managed
2.3 Misconception #3: A Supplement to Business Strategy
2.4 Quo Vadis?
3 STRATEGY
3.1 The Playing to Win Strategy Framework
3.2 What Strategy Actually Is
3.3 Plan vs. Strategy: The Typical Misunderstanding
3.4 The Strategic Choice Structuring Process
3.5 What Strategies an Organization Needs
3.6 Reinforcing Rods
3.7 What Strategies an Organization Doesn’t Need
3.8 Strategy Design Teams
3.9 Summarizing Strategy Fundamentals
4 DATA STRATEGY DEFINITION & DESIGN
4.1 Defining Data Strategy
4.2 Data Strategy Choice Cascade
4.3 Data Strategy Design Process
4.4 Step I: Identify Data & AI Demands
4.5 Step II: Collect Challenges
4.6 Step IV: Operational Capabilities & Systems
4.7 Step V: Maturity Assessment
4.8 Step VI: Planning
5 INTEGRATING BUSINESS STRATEGY & DATA
5.1 Data Choices as Part of Your Business Strategy
5.2 Data for Problem Formulation and Possibility Generation
5.3 Data Use Case Validation as Part of Testing
5.4 Concluding Remarks on Business Strategy & Data
6 PRACTICAL EXAMPLE
6.1 Step I: Identify Data & AI Demands
6.2 Step II: Collect Challenges
6.3 Step III: Designing a Data Strategy
6.4 Steps IV-VI: Activating Strategy
7 CONCLUSION
REFERENCES
1 THE FUZZ ABOUT DATA STRATEGY
Data, analytics, and Artificial Intelligence (AI) are not new phenomena in business but have grown increasingly relevant over the past decades.
A lot of excitement currently exists around generative AI due to its recent impressing advances for generating outputs such as texts or images. When I refer to AI, I do not mean solely generative AI, but the broader context of tools to generate value from data. And these tools include Business Intelligence, Machine Learning or Data Science and are everything but new. They have been around for many years.
Where does the persistent interest come from?
1.1 Data Can Create Benefits Throughout the Organization
Whilst data was once mainly relevant to accounting and controlling teams, it is nowadays often success-critical throughout the organization:
- Marketing uses historical campaign data to identify factors that drive success, enabling more effective allocation of marketing spend.
- Procurement uses data and analytics to analyze supplier performance, so that disruption can be minimized and cost savings maximized.
- Sales uses historical data to recommend an optimal point-of-sale portfolio leading to increased revenue.
- Customer service centers leverage data to predict customer churn, enabling proactive actions that reduce churn rates and drive sustainable revenue growth.
- Production departments leverage IoT data to prevent machine failures, reducing repair costs and minimizing production downtime.
- Product development teams enrich existing products and services with digital applications, such as delivering value-added insights to customers, increasing customer loyalty, and securing a competitive advantage.
Many organizations have been successfully leveraging value from data based on such analytical data use cases. But data and AI can do so much more.
Data and AI provide the means to question both, the status-quo of companies and entire industries. They allow organizations to gain a knowledge advantage by asking the right questions about customers, technologies, competitors and industry thus providing a mean to gain and sustain a competitive advantage. It is about combining data insights with human creativity to actively design the future [1a, 2].
Given the vast benefit potentials, it is no surprise that more and more organizations are eager to leverage data as a strategic asset. Companies strive to become data-driven — or rather data-inspired [2], which means that they aim to:
- Consistently leverage the potential of already existing data for optimizing their businesses
- Use data and analyses as integral part of their business innovation to rapidly test new creative ideas, which advances their thinking and increases competitiveness
So where is the catch?
1.2 Organizations Struggle to Become Data-Driven
Despite its promise, many organizations continue to struggle with leveraging the benefits of data, analytics, and AI. How is this possible after technology, algorithms and methodologies have been available for so many decades?
Becoming data-driven can be a complex endeavor
Organizations often underestimate the complexity of becoming data-driven, especially when the lack of strategic clarity and data literacy hinders progress.
A lot of things can go wrong when organizations start an endeavor to leverage data as an asset. It is not about buying some plug-and-play technology solution as many vendors tend to promise.
Becoming data-driven is often referred to as a data-driven business transformation [3]. It is called transformation for a good reason, as it typically involves changes that impact the entire enterprise.
For example, data needs to be acquired and integrated from various sources, so it can be made available for the business. The data integration requires company-wide orchestration and governance. Without it, each data consumer is left to repeat the tedious and error-prone work of data preparation, ultimately resulting in a ‘data wild west’ where different truths are reported, and valuable resources are wasted.
Leveraging data and AI is the responsibility of the business
The actual value generation with data mostly happens in the business units and functions, i.e. in Marketing, Procurement, Sales or Manufacturing.
Many assume that becoming data-driven is purely a matter of technical expertise in IT and analytics, overlooking the cultural and organizational changes it demands. But this is a fallacy.
Business users need to become data literate themselves. They need to embrace a culture of innovating, identifying and developing data use cases, which are relevant for the decision making in their area. They need to be able to use data to discover new insights that provoke new questions and spark innovative ideas to create competitive advantage.
Organizations require cultural and technical capabilities to become data-driven, but struggle to identify, build and maintain these.
Finally, leveraging data is a team effort and requires interdisciplinary stakeholders and thinking.
Data, analytics and AI are not the cure for everything
Data, analytics and AI are currently over-hyped and people seem to forget that the basis for each algorithm is mathematics. However, every statistical algorithm has underlying assumptions and inherent limitations. Relying solely on data for decision making is as bad as the opposite extreme, i.e. not using data at all for decision making.
The famous quote “prediction is difficult, especially when dealing with the future”, which originates from the Danish politician Karl Kristian [4], hints that you need to apply any forecasting with care: One underlying assumption of making predictions based on historical data is, that the underlying system, which generated the data will not fundamentally change in the future. This assumption can often be violated and a reputable data professional must carefully check it before applying fancy algorithms in a crystal ball fashion.
The fact that data, analytics and AI are over-hyped can be problematic, as stakeholders may have unrealistic expectations. This needs to be addressed, by establishing data literacy and culture in the organization fostering a common language and understanding leading to realistic expectations.
To provide an example, one particular field where analytics needs to be treated with care is the strategy design process, as the aim of an innovative strategy is often to uniquely shape the future with an impact on the market. If such a strategy is successful, a sales forecast based on sample data from the past might be unrepresentative and worthless to derive meaningful conclusions from it [1a, 1b].
The lack of business strategy as a root cause for frequent failure of data & AI
As I argued in the second article [5] of the Demystify Data Strategy article series, I believe that the majority of problems organizations have with managing and leveraging data as an asset stems from the lack of strategic groundwork. With strategic groundwork, I do not mean commonly used (mis-) conceptions of data strategy — as we consider below — , but genuine strategy design work which naturally integrates data-related strategic choices into business strategy.
Therefore, the present article extends this idea into a ready-to-use framework, leveraging tools of the well-known Playing to Win strategy framework.
But first, let’s have a closer look at the promise of data strategy and the misconceptions surrounding it.
1.3 The Data Strategy Promise
As the applications of BI and Data Science grew during the last decades, organizations increasingly struggled with managing the complexity of both: data management and the creation and scaling of analytical solutions. More structured approaches were needed and data strategy was and still is the supposed recipe to address these issues.
For writing this article I tried to find the origin of the term data strategy. Using the Google book search I found the book ‘Design and strategy for distributed data processing’ [6] from 1981.
Consequently, the term data strategy is far from new, but has gained increasing interest since 2010, as shown by a Google Trends [6] search, which matches with my personal experience as a data professional during this time period.
Strategy is generally perceived as something clever allowing to manage complexity and the promise of data strategy lies in addressing the challenges organizations face with leveraging data, analytics, and AI.
However, there is a catch: Despite its growing prominence, ‘data strategy’ remains poorly defined and is most often misunderstood, resulting in miscommunication, misaligned initiatives, and unmet expectations.
To understand why organizations continue to struggle with data strategy, we must first address the misconceptions surrounding the term.
2 DATA STRATEGY MISCONCEPTIONS
As a data professional and management consultant, I have learned that clear communication is critical for success. Clear communication begins with a common language, and this is where the problems with data strategy start: There is no consensus on what it actually means or should be. Data professionals, as well as business experts, use it in different contexts whilst meaning different things.
There is currently no universal agreement on what a ‘data strategy’ truly entails.
Furthermore, the most commonly used definitions of data strategy are incompatible with well-established strategy definitions. In my opinion this creates a severe problem, as it hinders target-oriented discussions of data professionals with executives and business stakeholders and is therefore a road-blocker for organizations to address their data challenges, preventing organizations to become data-driven.
The lack of joint understanding for data strategy hinders target-oriented discussions between business and data professionals and is therefore a road-blocker for organizations to leverage data as an asset.
This lack of consensus leads to widespread misconceptions about data strategy.
While many publications on data strategy deliberately or unintentionally avoid explicitly defining the term — assuming the reader already knows what it should mean — when definitions are provided, they often reveal misconceptions. Let’s explore three of what I think are the most common ones.
2.1 Misconception #1: A Plan to Build Data and AI Capabilities
Plans focus on execution and timelines, while strategies center on making deliberate choices under uncertainty. Conflating the two leads to confusion and inefficiency.
The Playing to Win strategy framework, is quite clear about the distinction between a plan and strategy [8] as we shall see in detail below in Section 3.3. However, when I google the term ‘data strategy’, several of the page one results — skipping the ads — define it as a plan or roadmap. Some examples are:
- “A data strategy is a long-term plan that defines the technology, processes, people, and rules required to manage an organization’s information assets.” [9]
- “A data strategy is a well-defined plan designed to improve the effectiveness of company-wide data management.” [10]
In essence, data strategy definitions that fall into this category, are about plans for building capabilities for data, analytics and/or AI. These organizational capabilities encompass elements such as data architecture, data governance, data management, BI, data science and data culture amongst others.
Defining data strategy as a plan to build data capabilities reflects a common misunderstanding of what strategy truly entails. Strategy should first define what data-related capabilities your organization truly needs.
A plan to build data, analytics and AI capabilities is not your strategy, but the output of your strategy work.
To create such plans can in itself be a challenging endeavor and their design is absolutely needed to leverage data as a company asset. So it is well invested time and requires solid competencies in a wide range of interdisciplinary fields. All I am saying is simply don’t call it data strategy, as it is what it is: a plan.
2.2 Misconception #2: Guidelines How Data is Managed
Another common definition frames data strategy as a kind of best practice guidelines clarifying how data is acquired, stored, managed, and leveraged.
Approaches for managing data are essential operational tools that support a strategy but are distinct from it. Referring to them as ‘strategy’ risks overshadowing the importance of deliberate, strategic choices.
How data is acquired, stored and managed are important topics and are mission critical to create a scalable data management foundation in any organization. However, approaches and guidelines should not be called strategy. They are just different things.
2.3 Misconception #3: A Supplement to Business Strategy
I must admit, early in my career, I too fell for the misconception that data strategy could exist as a separate supplement to business strategy. However, a data strategy should never stand apart, defining how data creates business value. This is inherently the role of the business strategy itself, as we will explore further in Section 5.
I like to visualize this using Lego blocks: the business strategy is an integrated set of interconnected Lego pieces, while a misconceived data strategy appears as a separate entity forcibly glued on top by the infamous ‘Lord Business’ with his dreaded ‘Kragle’ glue [11], stifling creativity and preventing innovative design.
What do I mean with data strategy as a supplement to business strategy? Whenever data strategy is defined as ‘something that defines how an organization will leverage data to realize its strategic objectives’, that is a misconception [1c]. A a well-defined strategy is singular [1d] with one business strategy for each indivisible business entity. The set of choices for the business strategy forms a harmonious overall whole. Trying to force something on top, such as a separate way of winning by leveraging data and AI, simply does not work. Therefore:
Having a supplementary data strategy that amends your business strategy by stating how your organization can use data, analytics, and AI to create business value and gain a competitive edge is not something your organization should aim for.
Instead, as I argued in part 2 of the Demystify Data Strategy series of articles [5], your business strategy should clarify if and how your organization uses data, analytics and AI to gain a strategic competitive advantage. A well-defined business strategy already includes how data creates value [1c]. Adding a supplementary data strategy dilutes focus and undermines integration.
Section 5 discusses a way to how to design a business strategy that leverages data strategically.
2.4 Quo Vadis?
To circumvent the first two of the three stated misconceptions for data strategy is relatively straightforward, as it is simply agreeing on a common language. Plans are plans, guidelines are guidelines, and strategy is strategy. These are distinct concepts, and both data and business professionals must recognize this in their communication.
But what about the third misconception, the fact, that there should not be a supplementary strategy for data?
What’s missing is a coherent definition of data strategy, that is understood from both, data professionals and business professionals alike.
To resolve this misconception, we need therefore two things:
- A coherent definition of data strategy that aligns with common business strategy definition and
- A process for integrating data-related choices into the fabric of business strategy
The remainder of this article will address this. It provides:
- A definition of data strategy, which is compatible with the definition of strategy in a business context
- Clarity on the role of business strategy for leveraging data as an asset
- A process for doing all the strategic work necessary to become data-driven
An important side effect of the suggested framework will be its positive impact on data leadership, contributing to the development of data culture and data literacy of executives.
The remainder of the article is structured as follows:
- Section 3 provides a summary of the renowned Playing to Win Strategy framework, which will serve as basis for all strategic groundwork required to become data-driven.
- Section 4 presents a definition of data strategy, that aligns and fits into the Playing to Win strategy framework. It also provides a detailed end-to-end process to conduct all the strategic work required for an organization to become data-driven.
- Section 5 clarifies, that designing a data strategy as defined in Section 4 alone will not be sufficient for an organization, that aims to become data-driven. You rather need to weave data-related strategic choices into the fabric of your business strategy thus blending strategy design and data use case innovation and development.
- Section 6 presents a detailed example of designing a data strategy for a salty snack food company that produces and sells potato chips and nuts. This section is intended for readers who wish to explore the data strategy design process in depth. Readers may skip this section without losing the overall flow of the article.
- Section 7 provides some concluding remarks.
3 STRATEGY
3.1 The Playing to Win Strategy Framework
Playing to Win is a strategy framework developed by Roger Martin [8], a globally recognized business thinker, advisor to CEOs, former Monitor consultant, and Professor Emeritus at the Rotman School of Management.
The framework was developed and continuously refined in the 1980s and 1990s [1e], and culminated in a joint book [13] by Roger Martin and A. G. Lafley, who is a former CEO of P&G. The book was published in 2013.
The framework became the standard strategy methodology at P&G and has since been successfully applied across numerous industries, establishing itself as a widely recognized approach to strategy. In addition, the Playing to Win framework has constantly been detailed by Roger Martin through an extensive series of Strategy Practitioner Insights [1, 1f].
While other strategy frameworks exist, I chose Playing to Win because it is widely recognized as a standard for effective strategy design. In addition, it comes with an entire ecosystem of resources such as literature, processes, templates and trainings that can be leveraged for designing any kind of strategy.
While Playing to Win provides a robust framework for designing business strategies, it also offers a way how data-related choices should be integrated into business strategy. This will be explored in detail in Section 5.
So, what is then a clear definition of the term strategy?
3.2 What Strategy Actually Is
Defining the term strategy
The Playing to Win framework for strategy design defines strategy as an integrated set of choices that form a plausible theory allowing an organization to win against its competition.
“Strategy is an integrated set of choices that uniquely positions a firm in its industry so as to create sustainable advantage and superior value relative to the competition.” [13]
The framework emphasizes “winning” to highlight that the ultimate goal of strategy is competitive success, not mere survival or participation in the marketplace. If you just play to play, you risk that at least one competitor figures out how to win and will ultimately push your company out of the market.
The Strategy Choice Cascade
The choices a strategy design team needs to make can be structured into five groups, which together build the so-called Strategy Choice Cascade, which is one of the core tools of the Playing to Win strategy framework.
I like to visualize the cascade using Lego pieces: Each box in the cascade represents a set of strategic choices — each choice symbolized by an individual Lego piece. The choices within each box are interconnected, and the boxes themselves reinforce one another, creating an integrated and cohesive strategy.
The cascade illustrates that an organization needs to make choices for:
- What winning means for the organization (Winning Aspiration)
- Which customers to serve and what products to provide (Where to Play)
- How the organization aims to win with these customers in the market (How to Win)
- What critical activities and resources it needs to realize this (Capabilities), and
- What systems, processes, norms, culture and metrics are required to ensure that these capabilities are built and maintained (Management Systems)
These choices are not meant to be a loosely-coupled list, but need to be well integrated to arrive at a compelling whole. This is your strategy.
Explicit vs. implicit strategy
If you think about it, every organization makes these five choices either explicitly and consciously in form of an articulated strategy or implicitly through its actions. E.g. an organization makes choices which products to sell and which not, or which customer to serve and which not.
As a result, every organization has a strategy — whether explicitly articulated or not. Such implicit strategy can be deduced from the actions a company takes [1g].
One problem with such implicit ‘strategy-in-use’ is that there might not be a consensus in the organization what the strategy is. This has two implications:
- It is difficult for the organization to improve as it is not clear where it currently stands.
- Actions based on it may be ineffective, as stakeholders act counterproductive.
Use cases for applying the cascade
The cascade is a tool that is not filled out just once during strategy design, but it is revisited and utilized repeatedly for various use cases throughout the strategy design process. For instance, it is used to:
- Reconstruct the strategy of your competitors by observing what they do: This is a nice practice to familiarize yourself with the framework and is a kind of icebreaker [1h] to get started with the strategy design process.
- Document your current strategy: This is a good way to build a common understanding of the status-quo and make your current implicit choices explicit, if this has not been done before.
- Document new strategic possibilities: The process for designing a strategy [1i] contains a creative element, where many possibilities for the future target strategy are generated and systematically evaluated. These are documented and detailed using the strategy choice cascade, one for each possibility.
3.3 Plan vs. Strategy: The Typical Misunderstanding
As the term strategy is associated with intelligence and purposefulness, it is used in a lot of situations for many things and activities. It is used to describe goals, mission and vision statements, business models, slogans or themes or any kind of initiative. If you want to make a term sound more fancy, just use strategy as a post-fix.
A list of typical strategy myths is provided in [1j], including the most dominant misunderstanding: A plan is not a strategy [8]. Roger Martin, the originator of the Playing to Win framework, spent a lot of time and energy to explain the difference between a plan and a strategy [8, 1j, 1k, 1l, 1m]. How do the terms differ?
Plans are about certainty. They lay out projects with timelines, deliverables, budgets, and responsibilities. Strategy is about uncertainty. It consists of choices formulating a bet how an organization aims to win.
Consequently, when most people say strategy, they actually mean a plan or a list of initiatives. However, these are completely separate things, although they go together: planning follows strategy. Once your strategy is designed and agreed on, you know the capabilities and systems needed to activate your strategy. To build these capabilities and systems, you craft plans.
This plan-strategy-confusion is also true for data strategy as we saw in Section 2.1.
Knowing what strategy is, how is it created?
3.4 The Strategic Choice Structuring Process
To design a strategy, it needs much more than simply filling out the five boxes. The second tool of the Playing to Win framework is an established process for strategy design, called the Strategic Choice Structuring Process.
This process is actually an all-purpose tool for addressing a gap between an actual and a desired outcome [1i]. It comprises seven steps.
Step 1: Problem Definition
Strategy is foremost a problem-solving tool [1n], so you first start with identifying your business need for strategy design. As your current strategy — implicit or explicit — is creating a problem, you require a new set of choices, that produce the outcome you desire.
Step 2: How Might We?
This step aims to produce a statement, that opens the discussion for the ideation phase in Step 3.
For the first two steps it is important to focus on the customer and not on internal elements.
Step 3: Possibilities Generation
This is one of the creative parts of the design process. It is about innovating different possibilities, how the problem identified can be addressed. For each possibility, a choice cascade is formulated.
Step 4: What Would Have to Be True?
In this step the strategy design team aims to uncover those conditions, that must hold, such that the possibility considered would make a great strategy. This exercise needs to be done for each possibility created.
The team asks what would have to be true for customers, the company, and competitors to uncover the underlying assumptions of each strategic possibility. It is important not to ask what is true, as this leads to unproductive discussions and the best strategies create a new future, that is, things that today are not true, might become true in the future due to the implementation of the new strategy.
Step 5: Barriers to Choice
Any condition identified in Step 4 can become a barrier if the strategy design team lacks confidence that it is true or can be made true in the future. An identified barrier can stop the design team to choose the corresponding possibility as final strategy in Step 7.
If a barrier has been identified, it needs to be tested.
Step 6: Testing & Transformation
For each barrier, a test needs to be designed and conducted to determine, whether the condition is currently true, or if there exists a plausible transformation path to make it true [1i]. This is the second creative element within the process.
The tests are not for achieving 100% confidence, which is impossible, but rather to shorten the odds for the strategic possibility, such that the design team is confident enough to pick it as the final strategy.
Step 7: Choice
After all strategic possibilities have been developed, potential barriers have been identified and tested, the strategy design team should have sufficient information to choose one possibility over others, which becomes the new strategy.
Note, that the Strategic Choice Structuring process contains elements of Design Thinking [1o] with phases of divergent thinking for Step 3 and Step 6, respectively [14].
3.5 What Strategies an Organization Needs
A company selling one product in one geography may only need one strategy [1d]. However, if business elements differ significantly in Where to Play and How to Win, each element will require its own strategy.
For each strategy, the above design process needs to be applied to arrive at a unique set of choices leading to a separate strategy choice cascade. Consequently, large organizations will have several strategy building blocks, and the challenge is to make them fit together and reinforce each other [1p, 1q, 1r].
“Strategy involves making choices under uncertainty, constraints, and competition, and that happens in lots of places across the company.” [1c]
Business strategies
The most core form for strategy is business strategy, which is the strategy for a service or product [1c] in one geographic region [1s]. At this indivisible level of strategy, an organization competes with given competitors for given customers segments.
Aggregation strategies
Another form of strategy is aggregation strategy [1q], which combines a set of strategies from a below level. The corporate strategy is simply the highest aggregation strategy within in organization. The task of an aggregation strategy is to add competitive value to the portfolio of businesses below, such that the whole is greater than the sum of its parts.
Typical nested aggregation levels are Corporate, Business Unit, Category and Brand. Another example for aggregation strategy is a regional strategy, such as for a given region or country.
Strategies for functions
A third form of strategy are functional strategies.
Functions are specialized areas within an organization, such as HR, IT, Finance, Marketing, and R&D, that provide support services to the broader organization or business units. They may operate as centralized teams (e.g., corporate functions) or decentralized units within specific business areas. The purpose of a function is to provide critical operational support and expertise to help the business units execute their strategies effectively.
Functional strategies are critical for ensuring that the specialized areas or teams within an organization deliver value to their internal customers while aligning with the organization’s overarching goals. Each function needs its own strategy [15] to determine:
- What services to offer to what internal customers
- How limited resources are prioritized
- How to achieve operational excellence while supporting the organization’s broader objectives
In addition to serving purely operational needs, some functions can play a strategic, integrative role by providing shared capabilities or systems that span across multiple business or aggregation strategies. These shared capabilities and systems are referred to as reinforcing rods [1q], which focus on creating synergies and align nested strategies across the organization. We will have a closer look at reinforcing rods in Section 3.6.
Strategy architecture
In larger organizations, where individual businesses have different competitors and customers, there exists an ecosystem of nested and inter-connected strategies.
Example
To illustrate the concept, I choose to depict a subset of strategy building blocks of a salty snack food company, that produces and sells potato chips and nuts.
There are two aggregation strategy layers: one for regions such as Germany and the UK and another one for the overarching corporate group level.
Below the aggregation strategy for the region Germany, there are four business strategies, each forming an indivisible unit, the base level where competition takes place. The nut brands epicure and healthy have different customers and competitors and need therefore a separate theory for winning, just as for private label nuts and potato chips.
The aggregation strategy for the German region provides reinforcing rods to leverage synergies provided by the Production and HR function. Each function requires its own strategy.
Where and how does a data strategy fit into the strategy architecture? In Section 4, I define data strategy as the strategy of the data function, situating it squarely within functional strategies. However, this alone is insufficient for an organization to become data-driven. As we will explore in Section 5, achieving this ambition requires more than just a well-designed functional data strategy — it necessitates embedding data-related choices into the individual business strategies, other functional strategies and the overarching aggregation strategies.
3.6 Reinforcing Rods
Similar to steel rods that strengthen concrete constructions, reinforcing rods are a concept in strategy that fortifies the overall strategic architecture. As discussed in Section 3.5, the primary task of an aggregation strategy is to add competitive value to the portfolio of businesses it spans. This is often achieved through reinforcing rods.
Reinforcing rods are capabilities or systems that span multiple business units or organizational levels, creating synergies that enhance value and competitive advantage. They strengthen the organization as a whole by linking different layers (e.g., corporate, business units, geographies) and enable them to perform better collectively than they would individually. In this way, reinforcing rods provide a net benefit to the indivisible strategies making them more effective.
Examples for reinforcing rods can be shared capabilities for R&D or brand building which are leveraged across regions.
Thus, reinforcing rods are the must-have capabilities or enabling management systems of an aggregation strategy. The aggregation strategy determines why these rods are needed and prioritizes them based on their strategic value to the organization. Their realization, however, is typically delegated to functions.
In summary, reinforcing rods are about building synergies with strategic impact across organizational levels, reducing duplication, and optimizing the use of specialized or scarce resources. They enable the organization to compete more effectively by ensuring that its nested strategies work together seamlessly.
3.7 What Strategies an Organization Doesn’t Need
Whenever you think you need a supplementary kind of strategy to amend or overlay your business, aggregation or functional strategy, it is likely a misconception. A strategy — by definition — is an integrated set of choices, which mutually reinforce each other. By overlaying a strategy with another one, you do not achieve integration, but the opposite, as you loose integration.
In [1c], Roger Martin provides examples for such undesired strategy degenerations. These are content strategy, partner strategy, growth strategy, sustainability strategy, digital strategy and data strategy.
As we already stated in Section 2.3, a data strategy as a supplementing entity to a business strategy is therefore a misconception that should be avoided.
The implications for this on our intention to help organizations to become data-driven to leverage data as an asset, will be discussed in Section 4 and 5.
3.8 Strategy Design Teams
A larger organization can have a set of different strategies, so who is in charge for what strategy design?
Typically, the leader of each entity that needs a strategy is ultimately responsible for making the corresponding strategic choices [1t]. This means the CEO is responsible for the corporate strategy, whilst the IT leader is responsible for the functional IT strategy and so on.
Strategy is rarely designed by a single individual, but it is rather a team exercise, where it is important to create engagement right from the start to activate the strategy [16]. However, this does not mean involving as many people as possible. The strategic decision-makers must possess the competencies to evaluate strategic choices in the context of competition, customers, and the company. These competencies should reside within the leadership team of the entity for which the strategy is being designed [1t].
Within a strategy design team, there typically exist the following roles:
- Sponsor: Responsible for overarching choices, advises and ultimately approves strategy
- Owner: The leader of the unit for which the strategy is designed holds ultimate responsibility for making strategic choices
- Core team member: People, that are responsible for jointly creating the strategic choices. For corporate strategy, this is the entire leadership team.
- Extended team member: People, that have a perspective, which is important to shape the strategy
- Subject matter expert: People, that hold a specific expertise
3.9 Summarizing Strategy Fundamentals
As we have seen, the Playing to Win framework provides a powerful and structured approach to designing strategies. It provides a rich ecosystem of definitions, tools and thinking that helps to generate any kind of successful strategy.
Strategy is not a plan, but an integrated set of choices that identifies a place to play and a theory for how to win there, that is backed by capabilities and corresponding management systems that build and maintain those capabilities [1d].
The Strategic Choice Cascade helps to structure the choices, which are necessary to be made. The Strategic Choice Structuring Process helps to design your strategy by starting with a problem formulating over generating and evaluating different possibilities until a final possibility can be chosen to be the new strategy to address the problem.
Now that we have a solid understanding of what strategy is and how it is designed, it is important to consider how data-related strategic choices fit within this framework. The misconception around data strategy — highlighted in Section 2.3 — can only be resolved by embedding data-related choices into the business strategy itself. This ensures that data, analytics, and AI are treated as enablers of strategic ambition, rather than as standalone or supplementary initiatives.
In the next section, we will explore how data strategy can be defined in a way that aligns seamlessly with the Playing to Win framework. In Section 5, we will then discuss how to ensure that the strategic demands for data are derived directly from the business strategy, creating a cohesive approach that integrates data use case innovation into the fabric of your organization’s overall strategy.
4 DATA STRATEGY DEFINITION & DESIGN
4.1 Defining Data Strategy
Most organizations require a dedicated function to provide data-related services or products, primarily to internal and eventually external data customers. This function requires a strategy as we saw in Section 3.5. This is your data strategy.
A data strategy is the strategy for the data, analytics, and AI function. It outlines how this function serves the organization’s broader business strategy by providing data-related products and services.
Your data strategy clarifies what winning means for the data function, who your internal data customers are, what services or products the data function provides, how it wins with its data customers, and what capabilities and systems are required to win.
Note that the data strategy does not define how data, analytics and AI create a competitive advantage for the organization. This is the task of your business strategy! In Section 5 I show, that data related strategic choices are a natural part of business strategy. The business strategy, in turn, provides the strategic data demands, the requirements for your data function, for which you design your data strategy.
Just as HR or IT strategies exist to serve the business, the data strategy is the functional strategy for delivering on data demands — not a standalone definition of how data creates value.
Failing to define a clear data strategy leads to a lack of focus, with the data function either trying to meet all demands (‘servile strategy’) or pursuing disconnected data initiatives (‘imperial strategy’) [15]. Both approaches undermine the function’s ability to win.
Depending on the nature and focus of the services and products provided in this function, you may call it data strategy, analytics strategy, BI strategy or AI strategy.
The strategy of such a function helps the data, analytics and AI teams to focus and is in my opinion the only entity which should be called data strategy.
4.2 Data Strategy Choice Cascade
When using the Playing to Win framework for the design of a data strategy, some terminology needs to be adjusted. This was topic of part one [17] of this Demystify Data Strategy article series. The adjustments we need, are:
- Offering → Data Offering: This includes products or services related to data, analytic or AI.
- Customers → Data Customers: People, who are served with the data offering. These are most likely to be internal stakeholders or groups within the company but can sometimes also encompass external stakeholders.
- Company → Data service provider: People, who create the data offering. Members of staff of the data function.
- Competition: Alternatives, that data customers might choose, other than the data service provider. This can range from data customers not served at all, serving themselves or using external services.
- Geography → Focus Areas: The business units, departments, teams, domains, or geographic areas your data strategy will focus on.
- Channels → Data Delivery Channels: How data customers get access to the data offering.
- Stages of production → Data Lifecycle Management: Which stages of the data lifecycle are done in-house or are outsourced.
After translating core elements, the Data Strategy Choice Cascade [17] can be defined as follows:
- Winning Aspiration: The definition of what winning looks like for your function.
- Where to Play: The playing field on which you will, and will not, choose to compete. This typically includes five dimensions: i) Focus Areas, ii) Data Customers, iii) Data Delivery Channels, iv) Data Offerings, v) Data Lifecycle Management.
- How to Win: The strategic advantage your data strategy will create. How you will win with data customers sustainably.
- Must-Have Capabilities: What critical activities and resources it needs to realize the How to Win.
- Enabling Management Systems: Infrastructure (systems, processes, governance, metrics & culture), which is needed in order to support and sustain your data strategy.
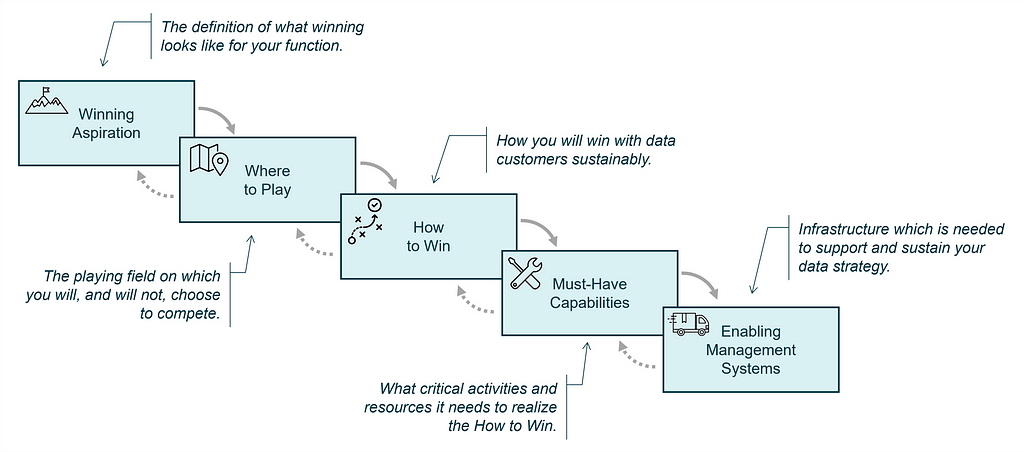
4.3 Data Strategy Design Process
As for any strategy design, we can apply the Strategic Choice Structuring Process of the Playing to Win framework. I propose five additional steps for developing a data strategy to incorporate the business strategy requirements and to explicitly distinguish it from the subsequent plan to build capabilities and systems.
The five additional steps for data strategy design are described in the following subsections.
4.4 Step I: Identify Data & AI Demands
From the above definition, it should be clear that a data strategy should not answer how data, analytics, and AI create business value and a competitive advantage. As I argued in [5], this should be part of your business, aggregation and functional strategies. These were discussed in Section 3.5. The strategic data demands for your data function are derived from these strategies and complemented with operational and eventual future data demands.
In essence, Step I is about gaining clarity about the existing requirements for data, analytics and AI in the organization. In total, there are three sources for such data demands, providing a natural prioritization for the subsequent data function to be built.
Prio 1: Strategic data demands
When working on your strategic architecture, the strategic data demands across the layers of your organization’s strategy should become clear as the individual strategy design teams progress. The inherent difficulty is that strategy design is not a linear process but an iterative going back and forth. This applies for the design of a single strategy [1u] as well as for the orchestration of different levels within a strategy architecture [1q].
Typically, strategic demands for data occur in different levels of your organization and may create the need for data, analytics and AI being a reinforcing rod of the strategic architecture (cf. Section 3.6). This can even be the motivator for designing a central data function as we will see in Section 6.
Another way, the strategic data demands can manifest themselves is in form of data use cases. We will encounter an example for such use case, which directly contributes to the competitive advantage of an organization later in Section 5.1. This use case can then stem the demand for a novel AI capability. Most often, this requires the business to be supported by a corresponding function for data and AI.
A further important aspect of strategic data demands concerns the desire of an organization to become truly data-inspired [2]. That is to constantly push its thinking by creating insights about customers, technologies, competitors and industry to gain competitive advantage. There might be a bit of recursion here, as such capability will also be used in the very heart of the business strategy design process itself, as we will see in Section 5. If an organization, however, fully acknowledges the enormous potential of data, which is not limited to boosting operational performance manifesting in a backlog of best-practices data use cases, this certainly is an requirement for designing the data function. This would lead to a Where to Play Choice within the data strategy, to support leadership with their business strategy design, as we will detail in Sections 5.2 and 5.3.
Ideally, the data demands should be actively stated by the corresponding strategy owner and arrive via choice chartering to a corporate data leader.
The best way for a data professional to collect the strategic data demands as input for a data strategy is to be part of the actual strategy design teams and actively help to innovate and evaluate strategic data use cases as we will discuss in Section 5.2 and 5.3. If this is not possible, you should analyze the current strategy architecture of your organization. If designed correctly, the individual strategic building blocks should directly provide the strategic data demands.
Unfortunately, in many companies strategy design appears to be a lost art [1v] and it requires a bit of detective work to figure out the current strategy architecture of the organization.
The set of identified strategic use cases across all strategy layers provides the most important requirements for data, analytics and AI, as the corresponding use cases are critical to support the organization’s strategy and thus competitive advantage.
Prio 2: Operational data demands
Data demands do not solely arise from strategic choices as part of strategy, but can also come from operational choices.
There are usually only a handful of strategic choices for capabilities and systems as part of your strategy. It is simply not the task of a strategy to provide an exhaustive list of organizational capabilities and systems, just those contributing to the theory of winning.
Thus, your strategic choices are typically complemented by other choices called operative imperatives [1w]. These are those operational choices which do not differentiate your organization from the competition, but are rather standard or best practices choices, positive things that everybody else is doing.
An example for such operational choice with data relevance might be capabilities for reporting and BI of a corporate strategy. It is best practice to have BI solutions for reporting in an organization, but these might not contribute to your theory of winning — they are just an operative imperative.
As with strategic data demands, another way operational data demands can manifest themselves is in form of data use cases. These operational data use cases provide point optimization such as efficiency gains of existing processes.
I typically discover the operational data needs through a series of interviews, which I conduct with business and data stakeholders of my clients.
Prio 3: Future data demands
In order to build a future-proof data strategy, an organization should consider a third category for data demands.
Often, different parts of the organization already have ideas or even plans how to leverage data and AI for their domain in the future. It is therefore important to identify these future data demands as these might have implications for the strategy of the data or AI function.
Such future data demands can be collected either in a passive way through interviews with business stakeholders or in a more active manner by conducting data use case innovation workshops.
Once all data demands are clear, you need to get a solid understanding of current pain points before diving into the data strategy design process as these might shape the problem formulation.
4.5 Step II: Collect Challenges
After clarifying the requirements for your data function, you need to identify potential challenges for leveraging data in your organization. These challenges will form the basis for selecting the problem definition in Step 1 of the Strategic Choice Structuring Process.
The task is to identify the most pressing deficiencies with data value generation in the organization. I typically include this as part of the interviews with business stakeholders when identifying the data demands in Step I. In fact, I usually ask for both, business-related and data related jobs, pains and gains. For documentation purposes, I like to borrow the right half of the famous value proposition canvas [18].
The outcome of this step is a solid understanding of most pressing data-related challenges, which together with the data demands provide a kind of fit-gap analysis as basis for Step 1 of the Strategic Choice Structuring Process.
As Step III of the data strategy design process is the standard Strategic Choice Structuring Process, which we already discussed in Section 3.4, I will skip a repetition here and directly jump to Step IV.
4.6 Step IV: Operational Capabilities & Systems
After Step III has been finalized, the data strategy is readily designed and needs to be activated [16]. This is done, by designing and building those capability and systems, which are required for the function to win. To build an effective data function, it is therefore essential to have a clear understanding of both, strategic and operational capabilities and systems required.
Strategic capabilities and systems are typically a small subset of capabilities and systems the data function requires. These are defined in your data strategy. Operational capabilities are those foundational activities, that enable the effective and reliable execution of strategic choices but do not directly contribute to winning with your data customers.
The distinction between strategic and operational capabilities and systems provides a natural prioritization where to invest most time and resources when building these elements.
As we saw, the operational data demands have been determined in Step I. Operational capabilities and systems are often directly derived from these. This could for instance comprise capabilities such as effective reporting or BI. Note that Step III.5 of the strategy design process, which is the Testing & Transformation step of the Strategic Choice Structuring Process, might provide the need for additional operational capabilities and systems.
Step IV is therefore to complete the picture by gaining an overview of what operational capabilities and systems the data function requires to succeed. For this, it might be helpful to make use of capability maps. Capability maps for data, analytics and AI are a well known tool for data professionals (cf. Figure 2 above) and are often used as a kind of checklist [3] when building data functions.
Typical elements for operational Enabling Management Systems in the context of a data strategy are:
- Data platform
- Data catalogues
- BI tools
- Decision frameworks
- Systems for developing a data culture
- Data maturity models
- Data Governance frameworks
- Skill matrices
- …
Once you have an overview about all strategic and operational capabilities and systems the data functions requires to succeed, it is time to assess the status-quo and to identify gaps.
4.7 Step V: Maturity Assessment
After the strategic and operational data capabilities and systems are defined, it is essential to assess your current state by conducting a data maturity assessment.
Many consultancies and data professionals tend to start with this type of activity early in the data strategy design process. However, I believe this approach is misguided. Whilst it is certainly valuable to have an overview what capabilities the organization currently possesses, a detailed assessment at the beginning of the strategy design process is of little value. You first need to identify the specific capabilities and systems required, along with the desired level of maturity for each. This means the set of capabilities and systems together with their respective target state must be defined. And this is, however, only possible after the data strategy has been designed.
Additionally, I am skeptical about data maturity assessments that focus on bench-marking against competitors or industry averages as I already argued elsewhere [19]. While such comparisons may seem insightful, they often provide limited actionable value for your organization, as they do not account for the unique strategic data demands and context of your business. Such maturity assessments rather pretend to be a standardized, simple solution, which does not live up to the complex problem of becoming data-driven.
However, a thorough current-state assessment of your capabilities and systems is needed as the basis for Step VI, where the plans to build and maintain each capability and system are created.
In addition, some organization successfully utilize data maturity models as a tool to measure their progress in data-driven business transformation programs and leverage it as a communication tool to various stakeholders in the organization. In these scenario, more complex metrics than simple traffic lights might be favorable.
4.8 Step VI: Planning
After performing a fit-gap analysis for each capability and system, it is time to craft a plan for building and maintaining the capabilities your data function requires to win. The plan to build data-related capabilities and systems is the topic most companies are enthusiastic about when it comes to data, analytics or AI. As we saw in Section 2.1, this is often falsely declared as data strategy.
Building each capability and system is typically a project in its own right, requiring an owner and a corresponding project team. The owner is ultimately responsible for designing and building the element.
The individual projects are often part of an overarching transformation roadmap for building a data-driven — or data-inspired — organization.
Implementing the corresponding projects gradually builds the operating model [20] for data, analytics and AI [21], which thus activates your data strategy.
Before we have a more in depth look at the data strategy design process using a practical example in Section 6, let’s consider how business strategy design and data use case design should be combined. This is required to determine your strategic data business needs, which is part of Step I above. We will discover this in the next Section.
5 INTEGRATING BUSINESS STRATEGY & DATA
In Section 4 we saw that it is not task of the data strategy — defined as functional strategy — to define how the organization creates value or a competitive advantage using data, analytics or AI. This is the task of the business, aggregation and/or functional strategies, which we defined in Section 3.5. These strategies define how to win. And sometimes, the organization can win with the help of data, analytics and AI.
In this section, we first have a look at how data strategic choices are a natural part of business strategy design. With data strategic choices I mean those choices of an organization’s theory to win, which rely on or involve the use of data.
I then argue, that data use case discovery, innovation and validation should be viewed as an integral part of the Strategic Choice Structuring Process for business, aggregation or functional strategy design. This naturally bridges business strategy and data use case design, which is often perceived as one of the greatest challenges for organizations to become data-driven.
5.1 Data Choices as Part of Your Business Strategy
When you make your choices of Where to Play, How to Win and what capabilities and systems you need to win as part of your business strategy design, some of these choices might rely on leveraging data, analytics and AI. Strategic choices for data and AI capabilities are a natural part of your business strategy. I provided some examples for this in [5].
Therefore, organizations should consider data and AI as something, which needs to be weaved into the very fabric of their business strategy.
Strategic choices with data or AI relevance are simply part of your business, aggregation, or functional strategy.
For illustration purposes, think of your strategic choices as Lego pieces, from which you build your Strategic Choice Cascade, and data-related strategic choices are just an integral part of it.
Let’s have a look at an example, where AI is a capability as part of the strategy.
Example
I used this example, which is borrowed from [1x], already before in part two of this series of articles [5]. It is about a salty snack producer, which uses a direct-store-delivery system, where the product is directly delivered to convenience stores and placed on the shelf by the delivery driver.
This direct-store-delivery system is labor-intensive and hence expensive, but it differentiates the company from its competition. It is a strategic choice for the regional aggregation strategy, those realization might be chartered [1r] to the Sales department.
Building data and AI solutions to predict store inventory and to generate optimal product orders for each point-of-sale helps reduce costs for the direct-store-delivery system. Hence, the choice to posses a data and AI capability, which enables the organization to predict store inventory, would be a strategic choice of the function Sales strategy, which directly supports the competitive advantage of the organization.
This data-related strategic choice provides a clear strategic data demand [5], i.e. a strategic requirements of the organization for the usage of data and AI. It is an integrated part of the company’s strategy and reinforces other strategic choices regarding differentiation from the competition.
The example illustrates that strategy — in this case it was the strategy of the Sales function — should define how data and AI create strategic value in the organization and not a supplementary data strategy ‘misconception’.
Data use case innovation should therefore be an integral part of business strategy design. The following two subsections show that the strategy design process of the Playing to Win framework provides a natural environment to integrate data use case innovation.
5.2 Data for Problem Formulation and Possibility Generation
Data use cases are the heart of data value creation, but organizations often struggle to identify their individual set of feasible and impactful use cases.
When innovating data use cases, it is often not differentiated whether the purpose is optimization of existing processes or the creation of new businesses opportunities. However, it is essential to differentiate between operational use cases, which address routine needs or improve efficiency within existing systems, and strategic use cases, which directly support competitive advantage and enable new ways of operating. The latter should be part of business strategy design.
There exist well-known and proven formats and techniques for use case discovery and innovation [2, 22]. These often incorporate elements of Design Thinking and are called Data Thinking [23], due to the inherent iterative approach required.
In fact, these formats and techniques can — and should — be directly integrated into the business strategy design process rather than being stand-alone exercises. This aligns nicely with the Strategic Choice Structuring Process, which is not surprising, as it also leverages Design Thinking [1o]. Thus, the Playing to Win framework allows to naturally combine data use case innovation and business strategy design, bridging the gap between business and data folks.
Integrating data use case innovation into the business strategy design process contributes to bridging the gap between business and data folks
This way, the business strategy design team can leverage data and AI as a tool to create strategic innovations by combining human creativity with data insights. This applies to Step 1 – problem formulation – as well as to Step 3 – possibility generation. Let’s have s look at an example.
Example
Consider the salty snack food company as in the previous examples. The salty snack food company noticed declining appeal among health-conscious consumers. An analysis of sales data, combined with social media sentiment analysis, highlighted a key insight: customers increasingly sought personalized snack options. Inspired by this insight, the strategy design team moved beyond merely optimizing existing offerings and stated the following problem with the corresponding how might we question:
1 Problem Definition
Customers are increasingly seeking personalized snack options, but our current product offerings are standardized, leading to declining market share among health-conscious consumers.
2 How Might We Question
How might we better meet the growing demand for personalized snack options among health-conscious consumers?
By combining the data-driven insights with creative formats, the team developed the following innovative possibilities:
3.a Possibility ‘Snack Kits’: Offer build-your-own snack kits in stores where customers can manually select ingredients to create their own mix, catering to their unique preferences.
3.b Possibility ‘Influencers’: Collaborate with health and wellness influencers to design branded snack bundles targeted at their followers, promoting personalization through co-creation with trusted figures.
3.c Possibility ‘Direct-to-Consumer Platform’: Design a direct-to-consumer platform where customers can input their dietary preferences and receive personalized snack bundles using AI-driven recommendations based on insights from customer data.
These possibilities weren’t simply derived from data — they were inspired by data, leveraging insights as a foundation for creative solutions that addressed both customer desires and business goals.
Furthermore, with possibility 3.c the design team created a new data use case. This illustrates that within the strategy design process, data and AI can be used to:
- Inspire the problem formulation
- Create new data use cases when innovating new possibilities
The implication for this is, that:
Subject matter experts for data, analytics and AI should be a natural part of any strategy design.
This connects nicely back to Section 4.4 and serves as a general motivation for including ‘business strategy design support’ as a Where to Play choice within the data offering of the data strategy.
Not only the innovation of new data use cases can be incorporated into the business strategy design, but also the subsequent iterative and incremental development.
5.3 Data Use Case Validation as Part of Testing
When organizations discover or innovate data and AI use cases, it is not always clear whether the desired solution will work as intended. There are uncertain assumptions that need to be identified, evaluated and eventually validated often using proof-of-concepts or minimum viable products. This is where design thinking meets data use case design.
This process perfectly aligns with Step 6 — Testing and Transformation — of the Strategic Choice Structuring Process outlined in Section 3.4.
The Strategic Choice Structuring Process naturally allows integrating iterative and incremental data use case development.
For possibility 3.c (Direct-to-Consumer Platform) of the previous example, this might look as follows:
Example (continued)
For each strategic possibility generated, the assumptions for the possibility being a great strategy need to be identified and evaluated. This is the task of Step 4 — What Would Have to Be True? — of the Strategic Choice Structuring Process.
Step 4 is about identifying critical conditions for customers, competition and company. To illustrate the concept, some of the critical conditions might be:
For customers, it would have to be true, that health-conscious consumers are willing to use a digital platform to personalize their snack options.
For competitors, it would have to be true, that they are either unable or unwilling to replicate the direct-to-consumer platform quickly. This is the so called can’t/won’t test [1x, 1y, 1z].
For company, it would have to be true that we can consistently derive meaningful and actionable recommendations from historical consumer data to personalize snack bundles effectively.
If the strategy design team would not be reasonably confident about that any of the identified critical conditions is currently true or could be made true, it would declare it as a barrier, which might stop the team from choosing possibility 3.c as final strategy. If a barrier has been identified, the team would design and conduct a test, in order to learn more and to gain (or lose) confidence that the condition could be made true. This is Step 6 of the Strategic Choice Structuring Process.
For the last of the three critical conditions above (company), a meaningful test could be to design a minimum viable product, where a data science team builds an algorithm using data available. The recommendations are then probed with a set of potential customers.
Such a test would validate, if the company has sufficient data to make meaningful predictions and if the data quality of that data as well as the prediction accuracy of the used algorithms are good enough to derive meaningful recommendations.
The example illustrates, that:
The data use case innovation and development process applied by data professionals, can be seamlessly integrated into the generic strategy design process of the Playing to Win framework.
Note that by doing so, business experts and data experts work together closely to design and probe new strategic possibilities, which addresses a common problem in organizations: the existing gaps between business and data teams.
5.4 Concluding Remarks on Business Strategy & Data
To conclude this section, we first link the strategic data demands originating from the business strategy design process back to the data strategy design process described in Section 4.3.
Strategic Data Demands as Inputs for Data Strategy
We saw that a part of Step I of the data strategy design process is to determine the strategic data demands of the organization, which then become the input in form of requirements for building a data function.
From this one may conclude that:
A solid design of business, aggregation and functional strategies is a necessary condition for success with data, analytics and AI in an organization.
And this is where in my opinion the root cause of why organizations fail with data & AI lies [5]: With solid strategy design becoming a lost art [1v], many organizations lack a sound strategic architecture and strategy design process. The lack of strategic competencies has many negative effects for an organization. One such effect is that organizations struggle to use data as an asset.
By leveraging the Playing to Win strategy framework to create a solid strategy architecture, organizations can embed data-related strategic innovation and resulting choices into their business strategy, generating clear strategic demands that inform and guide the design of the data function’s strategy, ensuring alignment and focus.
Data leadership
As we concluded that leveraging strategic value from data, analytics and AI is the task of business strategy design, it is clear that the responsibility lies ultimately with the respective strategy owners, i.e. the corresponding leaders.
This emphasizes once more that leaders of all kinds and levels need to embrace a data culture, ensuring they understand, are willing to, are skilled to, and are required by their superiors to exploit possibilities to use data and AI as strategic levers. They need to become so called ‘data explorers’ as defined in [2].
Leaders of all kinds need to become data explorers
Data, analytics and AI are therefore a score skill in a digital world. That does not solely apply to data professionals such as data scientist or data engineers, but is also true for business leaders.
The ROI question for programs to become data-driven
Transformations of any kind are not an end in themselves. A transformation program to become data-driven should always arise from business strategy. Such programs are the plans and initiatives designed to activate the strategy, ensuring the realization of the organization’s Winning Aspiration, Where to Play, and How to Win choices.
When it comes to committing resources to these transformation programs, I often encounter executives and leaders raising questions about the return on investment (ROI) — an understandable concern. However, the evaluation of anticipated costs should occur earlier during the business strategy design process, not during data strategy design or even at the implementation stage.
Ideally, the need for specific capabilities or systems should naturally emerge from a well-defined strategy. When ROI calculations dominate the discussion, it may indicate that the leadership perceives the proposed investments as unaligned with immediate strategic priorities.
During the business strategy design process, the design team should ask, “What would have to be true regarding the cost of building the required capabilities and systems?” If uncertainty exists about the costs, this critical condition becomes a barrier, prompting the need for testing. Such tests must evaluate both the strategic benefit and the associated costs, ensuring that data investments are assessed within the broader context of organizational priorities.
This again highlights the importance of involving data teams in the business strategy design process and demonstrates that strategy and execution are not separate phases but instead form a seamless transition to activation as soon as capabilities and systems are designed.
The next section provides a detailed walk-through of the developed framework, applying it to a practical example that builds upon the earlier case of a salty snack company.
6 PRACTICAL EXAMPLE
In Section 4.3, we introduced an amended version of the Strategic Choice Structuring Process for designing a data strategy. In Section 5, we demonstrated how data-related strategic choices are integral to the business strategy, forming a key requirement for designing the data function.
Now we have all jigsaw pieces together to do the strategy work which is required in an organization to leverage data as an asset using analytics and/or AI.
This section provides a detailed example, illustrating how a data strategy can be developed. For this, we use again the salty snack food company, that produces and sells potato chips and nuts, for which we design a strategy for a group-wide data function. The section is meant to be a deep-dive and can be skipped without losing too much context.
Recall, that we touched upon this example already in Section 3.5 and Sections 5.1, 5.2 and 5.3. We will now go through the steps of the data strategy design process depicted in Figure 11.
6.1 Step I: Identify Data & AI Demands
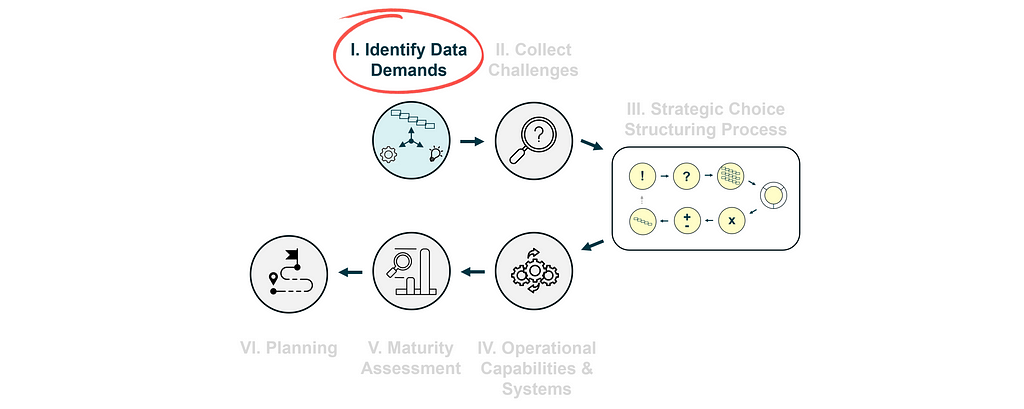
Strategic demands
As part of the group strategy design, the salty snack food company chose to add competitive value to the country businesses by building a new reinforcing rod. The possibility chosen entailed to centralize all major IT systems (Enterprise Resource Planning, Customer Relationship Management, Manufacturing Execution System), leading to enabling management systems that span across the entire organization.
Thus, the multinational salty snack food company decided to undergo a major IT harmonization program with the goal to centralize all systems and corresponding business processes across all countries.
Based on this, the group strategy design team also chose to build another reinforcing rod for data, analytics and AI, which should be realized through a newly group-wide data function. For instance, this entailed the harmonization of all reporting solutions for the individual countries.
From several regional Sales function strategies, another strategic demand was to provide solutions for store inventory prediction, as we saw in Section 5.1.
These strategic data demands were directly tied to the organization’s strategy, ensuring that the data function supports the competitive advantage of the organization. Strategic demands took precedence as they directly support the organization’s theory of winning, while operational and future demands addressed foundational and exploratory needs. Let’s have a look at the latter two.
Operational demands
A critical operational data demand for the new data function was to ensure that the harmonized systems and centralized reporting solutions were designed to seamlessly replace the existing local systems at the rollout time, without interrupting day-to-day operations. During the transition phase, the existing local data teams would be responsible for maintaining the continuity of current operational dashboards and reporting solutions (e.g. for sales tracking, inventory and production management).
Future data demands
Whilst preparing for the forthcoming data strategy design process, the design team discovered several future data demands through a series of interviews with the individual regional units.
One such future data demand was the planned digital manufacturing program in several producing countries, which aimed to optimize production and quality by intensively leveraging digital and data potentials. The program’s roadmap included potential data use cases, such as predictive process tuning for production machinery.
Strategy Design Team
The team for the designing the data strategy consisted of representatives from both the corporate data function and regional stakeholders.
At the corporate level, the team included the group data leader, who owned the data strategy as she was in charge of the group data function. Other members of the core team were a data governance specialist and an analytics manager overseeing centralized reporting frameworks. As the group CEO chartered the choice of building the reinforcing rod, he acted as sponsor for the data strategy design.
From the regional perspective, the core team involved the German division’s data manager, which oversees the German data, analytics and AI demands and solutions. Furthermore, a representative from the manufacturing function was part of the core team to ensure alignment with local needs, such as digital manufacturing initiatives.
In addition, external subject matter experts for strategy design, data and culture where leveraged. This ensured a sound methodical approach and allowed to address change-related challenges form the very start.
The extended team consisted of several analytics power users and stakeholders with key requirements, who were involved on demand.
This composition allowed the team to balance corporate priorities with the specific demands of regional teams.
6.2 Step II: Collect Challenges
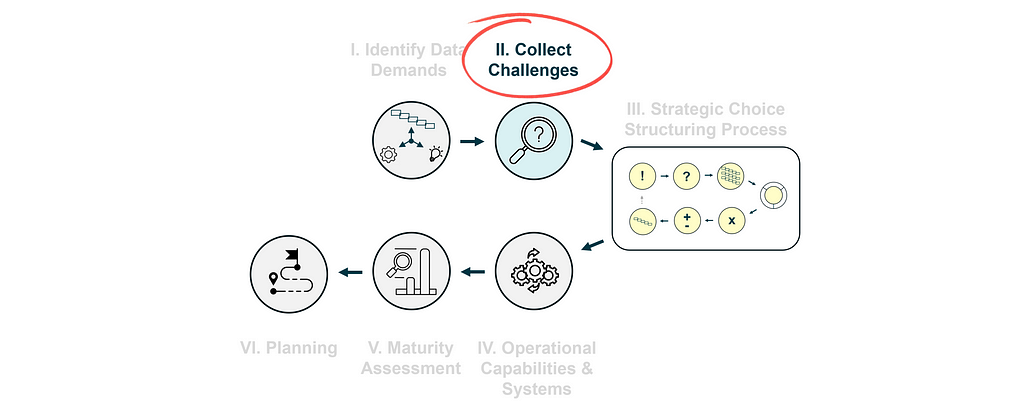
During the preparation phase, interviews with stakeholders across the group and regional units uncovered the most pressing challenges related to data, analytics and AI. These challenges needed to be addressed during data strategy design, in order to ensure the successful implementation of the centralized data function while minimizing disruptions and maximizing the value of the group data function.
The following key challenges were identified:
1 Uncertainty about future state
- Regional units voiced concerns about the clarity of the target operating model for the group data function, especially regarding their role and autonomy in managing and leveraging data after the rollout.
- Stakeholders highlighted a lack of understanding of how the harmonized systems and reporting solutions will meet both group-level and region-specific requirements.
2 Resistance to centralization
- Several regions raised concerns about losing flexibility and responsiveness in addressing their unique data business needs, particularly in scenarios where ad-hoc reporting or rapid adjustments were required.
- Fear of a one-size-fits-all solution failing to meet local business nuances was cited as a potential hurdle to adoption.
3 Skills and capability gaps
- Regional teams highlighted a need for training and support to adapt to the new centralized systems, particularly for self-service analytics and AI use cases.
- A skill and capability gap of the local business was identified in leveraging predictive analytics use cases, such as those outlined in the digital manufacturing program.
4 Interim business continuity risks
- Maintaining uninterrupted operational reporting during the transition phase remains a top priority for local teams, as any disruptions could impact critical business decisions in areas like sales, production, and inventory management.
- Stakeholders highlighted challenges in meeting local interim demands for data and analyses. They expressed uncertainty about which new local solutions would be worth developing within the existing legacy environment prior to the roll-out and which solutions could be deprioritized.
5 Alignment with Future Programs
- The design team noted that the success of future programs, such as the digital manufacturing initiative, would depend on establishing a robust data infrastructure and architecture that is flexible enough to support emerging data use cases like predictive process tuning.
6.3 Step III: Designing a Data Strategy
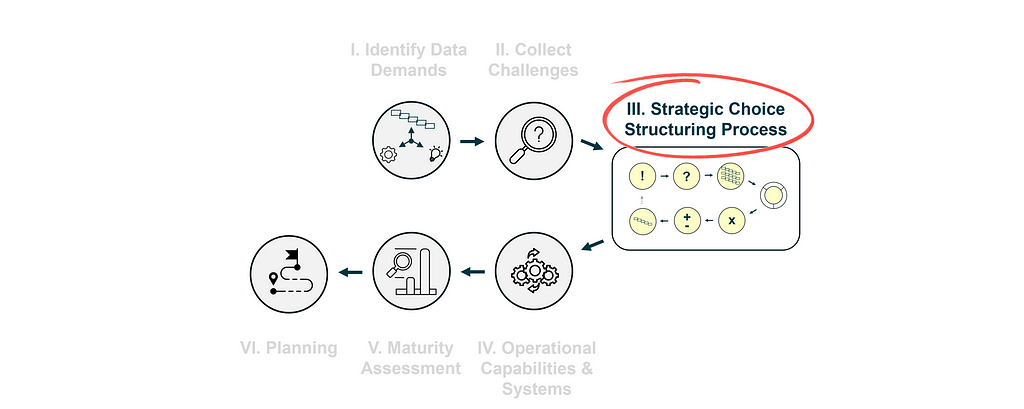
The strategy design process followed the Strategic Choice Structuring Process depicted in Figure 7, which itself consists of 7 steps.
Step 1: Problem Definition
The data strategy design team began by defining the primary problem they aimed to solve. The team defined the problem as:
“We need to harmonize data, analytics, and AI services across all regions based on the newly centralized IT landscape, while meeting diverse regional needs, maintaining business continuity, and enabling future data-driven opportunities.”
Step 2: How Might We?
The how might we question was designed to inspire innovative possibilities for the data function’s strategy while keeping the focus on delivering value and addressing the core challenges.
“How might we create value for our regional units through harmonized data, analytics, and AI solutions, while addressing their unique needs and aligning with our centralized IT landscape and governance?”
Step 3: Possibilities Generation
After defining the problem and framing the “How Might We” question, the team generated strategic possibilities.
For illustration purposes, I have limited the possibilities of the example to two. These possibilities contrast distinct approaches to structuring and operating the function, focusing on the degree of centralization and regional autonomy. Typically, teams may develop up to six possibilities and may contrast other aspects such as the level of relying on external professional service companies or how offensive or defensive the strategy should be [14].
Possibility ‘fully centralized data function’
This possibility envisioned a fully centralized governance and operational model for the data function. All major data, analytics, and AI activities, including data integration, governance, demand management, and solution delivery, would be managed by the central team. Regional units act as data consumers, relying on standardized reporting frameworks, dashboards, and analytics solutions provided uniformly by the central team to ensure consistency, scalability, and efficiency.
Possibility ‘federated data function with regional customization’
This possibility proposed a federated model that combines centralized governance with significant autonomy for regional units. Regional teams would play an active role in demand management, analytics development, and leveraging data science/AI for local initiatives, particularly in programs like digital manufacturing.
Choice Cascades
To detail both possibilities, the team completed the Data Strategy Choice Cascade for each. This helped the team to build a detailed joint understanding of each possibility.
Fleshing out the Must-Have Capabilities and Enabling Management Systems was for the design team already the first step to activate the data strategy as it was a kind of reality check for each possibility.
Step 4: What Would Have to Be True?
In order not to blow up the content of this section, I will only focus on possibility B for steps 4–6 of the Strategic Choice Structuring Process, as it will be sufficient to illustrate the concept.
The critical conditions were collected for customer, company and competition.
Recall the translations for company and competition we defined in Section 4.2 for this exercise.
Customers
- Regional data customers value the ability to create their own data solutions to meet their country-specific needs
- Regional data customers have the competencies to tailor analytics and AI solutions to their specific needs through locally available tools and experimentation.
- Regional business units trust that centralized governance frameworks enable, rather than hinder, their ability to innovate and adapt to local needs.
Company
- The central data team is able to provide effective governance frameworks and tools that empower regions to innovate while maintaining alignment with group-wide standards.
- Regional teams successfully develop and share innovative use cases and best practices that are evaluated by local teams and adopted across other regions where sensible.
- Resources are allocated effectively to balance support for both central governance and regional customization, without overburdening either side.
- The company can implement and maintain the federated model in a cost-effective way, ensuring resource allocation supports both central and local capabilities.
Competition
- The federated model outperforms do-it-yourself workarounds (e.g. with Excel) or external consultancies in delivering faster, more integrated, and cost-effective analytics and AI solutions.
Step 5: Barriers to Choice
From these critical conditions, the data strategy design team was least confident about two:
Customer-3: “Regional business units trust that centralized governance frameworks enable, rather than hinder, their ability to innovate and adapt to local needs.”
The reason for the low confidence was, that regional business units have historically relied on local data experts or external consultants for data solutions. It was unclear whether the data consumer would accept the new central authority.
Company-4: “The company can implement and maintain the federated model in a cost-effective way, ensuring resource allocation supports both central and local capabilities.”
The reason for the low confidence was, that the team had at the time not enough knowledge about the costs of both, data platform and tools as well as staff costs as the overall data operating model was not detailed to a sufficient extent.
Step 6: Testing & Transformation
To address these uncertainties, the data strategy design team developed a series of tests to validate the two critical conditions.
To address the identified barriers, the data strategy design team devised two focused tests:
Test 1 (trust in new data governance model)
The design team created a rough sketch of the future data governance operating model, that made the implications for local data consumers more tangible. The draft was shared with local key stakeholders and their feedback was incorporated within a next design iteration of the data governance operating model.
Test 2 (cost efficient implementation)
The design team created a first draft of the data operating model detailing the technological solution to estimate the future total cost of ownership for technology. They also detailed the newly required expert roles to estimate the future staff cost of the data function to be built.
These tests provided actionable insights into the assumptions and validated the possibility’s potential to succeed.
Step 7: Choice
In the context of this example, the data strategy design team decided to pursue the federated model, as it offered the highest likelihood of winning with the data customers by better balancing the need for regional innovation and customization while achieving the group’s centralized governance and alignment goals.
6.4 Steps IV-VI: Activating Strategy
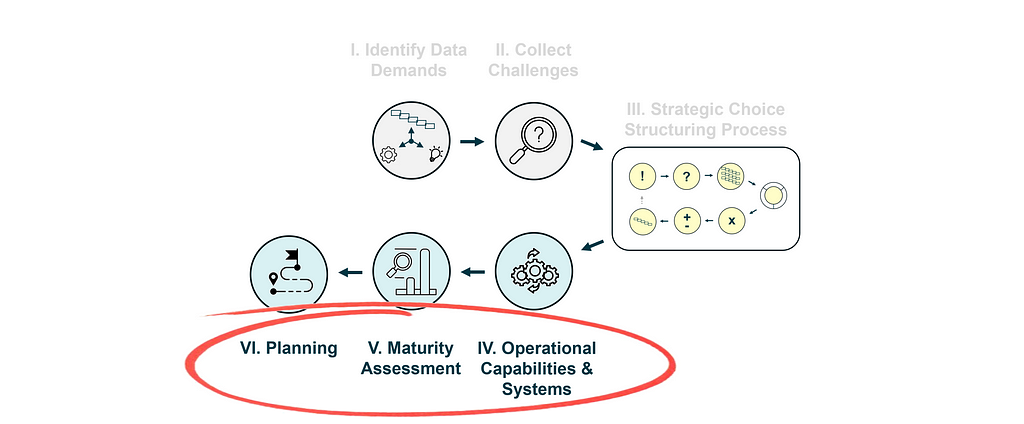
Once possibility B was selected as the new data strategy, the next task was to activate it by addressing the implementation requirements. The steps IV to VI ensured that the strategic choices made during the design process were translated into operational realities, enabling the data function to deliver on its strategic demands. By activating the data strategy in alignment with the business strategy, the organization ensured that data capabilities directly support its competitive goals.
Step IV: Determining operational capabilities & systems
After the strategic capabilities and systems had been defined in Step III as part of the data strategy, the design team identified the operational capabilities and enabling management systems required to implement the federated model effectively.
Key operational capabilities included efficient report generation. These were complemented by operational systems such as BI and ETL tools as well as systems to monitor data quality.
Step V: Data Maturity Assessment
To assess readiness for the data strategy, the team conducted a comprehensive data maturity assessment for all capabilities and systems required to activate the strategy and across all regions.
They used a traffic light evaluation metric to assess current capabilities and systems in areas such as data modeling, reporting, and local experimentation. Capabilities and systems with “green” ratings were deemed ready to adopt the data strategy, while those flagged as “yellow” or “red” required targeted interventions in form of dedicated projects to build and maintain the new capabilities and systems.
The assessment also revealed regions where experimentation and self-service adoption were underdeveloped, providing a roadmap for prioritizing maturity-building efforts.
Step VI: A Plan to Build Data, Analytics and AI Capabilities
Based on the maturity assessment findings, the team developed a phased plans to build and sustain the required capabilities and systems with priority assigned to those elements of strategic nature.
Each region was assigned specific milestones tailored to its maturity level, with clear timelines and success metrics. To ensure continuous improvement, feedback loops were integrated into the plan, enabling the central team to refine tools and frameworks based on regional experiences and evolving needs.
7 CONCLUSION
This article aimed to demystify data strategy by addressing common misconceptions that prevent organizations from fully leveraging data, analytics, and AI.
Contrary to popular belief, a data strategy is not a plan for building data capabilities, a set of guidelines for managing data, or a supplementary element to business strategy. Instead, it is a functional strategy — the strategy for the data, analytics, and AI function, which provides data services to internal customers.
The data strategy is designed on the basis of the organization’s overarching business strategy. And it is business strategy, not data strategy, which defines how the organization leverages data to achieve competitive advantage.
By leveraging the Playing to Win framework, I demonstrated that data-related strategic choices must be embedded within the organization’s business strategy, forming the input for a robust data strategy. By integrating data use case innovation into the business strategy design process and deriving clear strategic demands, organizations ensure that their data strategy serves as a functional enabler, delivering capabilities that directly support competitive advantage.
Using the practical example of a salty snack food company, I illustrated how organizations can apply this approach to bridge the gap between theory and practice. The structured process not only aligns the data function with business needs but also allows data to become a key driver of competitive advantage.
Organizations that continue to treat data strategy as a standalone supplement or a list of technical initiatives will fail to realize its true potential. Instead, by weaving data-related strategic choices into the very fabric of their business strategy, they can build a cohesive, purpose-driven strategy architecture that enables them to win.
The promise of data, analytics, and AI lies not in their technical complexity but in their strategic and cultural alignment. By embedding data innovation into business strategy design, organizations can move beyond the data hype and leverage data as a true strategic asset.
I welcome your feedback, suggestions, and questions. Do you agree, disagree, or have additional thoughts? I look forward to engaging in discussions with you in the comments here on Medium.
REFERENCES
[1] Roger Martin, Playing to Win/ Practitioner Insights (2024), website with list of articles
[1a] Roger Martin, What Strategy Questions are You Asking? (2023), Medium article of the ‘Playing to Win Practitioner Insights’ series
[1b] Roger Martin, Overcoming the Pervasive Analytical Blunder of Strategists (2021), Medium article of the ‘Playing to Win Practitioner Insights’ series
[1c] Roger Martin, Strategy, Strategy Everywhere (2023), Medium article of the ‘Playing to Win Practitioner Insights’ series
[1d] Roger Martin, Strategy is Singular (2023), Medium article of the ‘Playing to Win Practitioner Insights’ series
[1e] Roger Martin, The Origins of Playing To Win (2023), Medium article of the ‘Playing to Win Practitioner Insights’ series
[1f] Roger Martin, (Playing to Win) x 5 (2024), Medium article of the ‘Playing to Win Practitioner Insights’ series
[1g] Roger Martin, Strategy is what you DO, not what you SAY (2020), Medium article of the ‘Playing to Win Practitioner Insights’ series
[1h] Roger Martin, The Best Strategy Icebreaker (2024), Medium article of the ‘Playing to Win Practitioner Insights’ series
[1i] Roger Martin, The Strategic Choice Structuring Process (2024), Medium article of the ‘Playing to Win Practitioner Insights’ series
[1j] Roger Martin, The Five Deadliest Strategy Myths, Medium article of the ‘Playing to Win Practitioner Insights’ series
[1k] Roger Martin, Why Planning Over Strategy? (2022), Medium article of the ‘Playing to Win Practitioner Insights’ series
[1l] Roger Martin, Strategy vs. Planning: Complements not Substitutes (2024), Medium article of the ‘Playing to Win Practitioner Insights’ series
[1m] Roger Martin, From Strategy to Planning (2021), Medium article of the ‘Playing to Win Practitioner Insights’ series
[1n] Roger Martin, Strategy as Problem-Solving (2020), Medium article of the ‘Playing to Win Practitioner Insights’ series
[1o] Roger Martin, Strategy & Design Thinking (2020), Medium article of the ‘Playing to Win Practitioner Insights’ series
[1p] Roger Martin, The Secret to Knitting Strategy Together Corporate-Wide (2021), Medium article of the ‘Playing to Win Practitioner Insights’ series
[1q] Roger Martin, Corporate vs. Business Unit Strategy (2022), Medium article of the ‘Playing to Win Practitioner Insights’ series
[1r] Roger Martin, Strategic Choice Chartering (2020), Medium article of the ‘Playing to Win Practitioner Insights’ series
[1s] Roger Martin, Understanding the True Building Blocks of Corporate Strategy (2022), Medium article of the ‘Playing to Win Practitioner Insights’ series
[1t] Roger Martin, Who Should Do Strategy? (2023), Medium article of the ‘Playing to Win Practitioner Insights’ series
[1u] Roger Martin, Overcoming the Integrative Strategy Challenge (2024), Medium article of the ‘Playing to Win Practitioner Insights’ series
[1v] Roger Martin, The Lost Art of Strategy (2021), Medium article of the ‘Playing to Win Practitioner Insights’ series
[1w] Roger Martin, Is or Is Not The Opposite Stupid on its Face? (2024), Medium article of the ‘Playing to Win Practitioner Insights’ series
[1x] Roger Martin, Distinguishing How-to-Win from Capabilities in Your Strategy Choice (2021), Medium article of the ‘Playing to Win Practitioner Insights’ series
[1y] Roger Martin, Can Your Strategy Pass Its Most Important Test? (2021), Medium article of the ‘Playing to Win Practitioner Insights’ series
[1z] Roger Martin, Untangling Value Proposition & Competitive Advantage (2022), Medium article of the ‘Playing to Win Practitioner Insights’ series
[2] Sebastian Wernicke, Data Inspired (2024), book in German language published by Vahlen
[3] Caroline Carruthers and Peter Jackson, Data Driven Business Transformation (2019), book published by Wiley
[4] Quote Investigator, Quote Origin: It’s Difficult to Make Predictions, Especially About the Future, website accessed on Jan. 4th 2025
[5] Jens Linden, The Root Cause of Why Organizations Fail With Data & AI (2024), Medium article published in Toward Data Science
[6] James Martin, Design and strategy for distributed data processing (1981), book published by Prentice-Hall
[7] Google Trends, Worldwide interest of the term ‘data strategy’ (2024), website
[8] Roger Martin, A Plan Is Not a Strategy (2022), video
[9] AWS, What is a data strategy? (2024), website accessed on Dec. 22nd 2024
[10] SAP, What is a data strategy? (2024), website article accessed on Dec. 22nd 2024
[11] Wikipedia, The LEGO Movie (2014), WIKI entry
[12] Roger Martin’s website (2024)
[13] A. G. Lafley and Roger L. Martin, Playing to Win (2013), book published by Harvard Business Review Press
[14] Michael Goitein, How a leading strategy expert combined Design Thinking and scientific inquiry to allow anyone to create winning strategies, blog entry accessed on Dec. 25th 2024
[15] Roger Martin, Jennifer Riel, The One Thing You Need to Know About Managing Functions (2019), article published in Harvard Business Review
[16] Jennifer Riel, How to Make Your Strategy Real (2024), IDEOU Blog entry
[17] Jens Linden, The Data Strategy Choice Cascade (2024), Medium article published in Towards Data Science
[18] Alex Osterwalder, Achieve product-market fit with our brand-new Value Proposition Canvas (2012), website accessed on Dec. 30th 2024
[19] Jens Linden, Unser Datenreifegrad ist „Reactive“ oder“Managed“ — und nu?! (2023), LinkedIn blog entry in German language
[20] Andrew Campbell, Mikel Gutierrez, Mark Lancelott, Operating Model Canvas (2017), Book published by Van Haren
[21] Jens Linden, Data & AI Operating Model Canvas (2023), LinkedIn blog entry in German language
[22] Datentreiber, The Datentreiber Method: Data & AI Business Design (2024), website accessed on Dec. 30th 2024
[23] Wikipedia, Data Thinking (2024), website entry on accessed on Dec. 30th 2024
[24] Leandro DalleMule, Thomas H. Davenport What’s Your Data Strategy? (2017), article published in Harvard Business Review
Unless otherwise noted, all images are by the author.
All Lego illustrations have been created with MECABRICKS.
I used ChatGPT 4o as sparring partner, to generate some of the example ideas.
How Most Organizations Get Data Strategy Wrong — and How to Fix It was originally published in Towards Data Science on Medium, where people are continuing the conversation by highlighting and responding to this story.
What's Your Reaction?
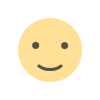
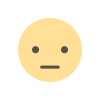
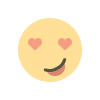
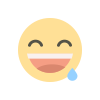
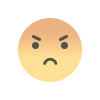
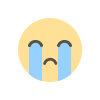
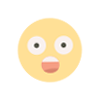